Barcoding Brains
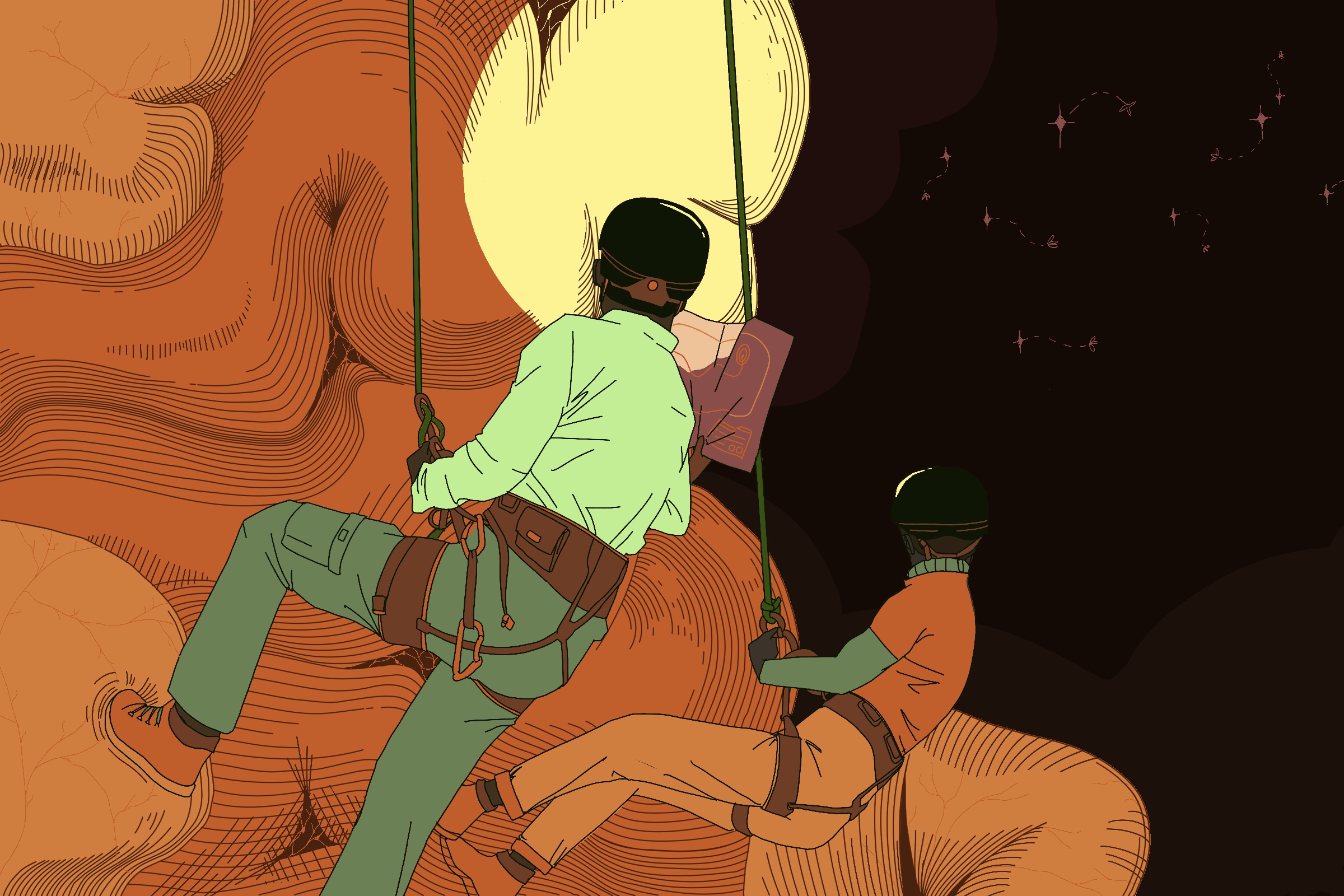
On the afternoon of September 13th, 1848, a metal tamping rod sailed through Phineas Gage’s skull at dozens of miles an hour before coming to rest yards behind him in the Vermont leaf litter. En route, it obliterated Gage’s left frontal lobe, a few molars, and his personality, leaving him “no longer Gage” to those who knew him well. Amazingly, Gage not only survived but is remembered as “the man who began neuroscience.”
Indeed, Gage’s case initiated a standard practice in neuroscience in which diseased or damaged brains are used to examine the connection between behavior and neuroanatomy — a method referred to as “lesion studies.” Gage, who, prior to his accident, had been temperate, hard-working, and pious became erratic, volatile, and irreverent. The connection between the destroyed lobe and Gage’s reduced capacity for emotional regulation was stark.
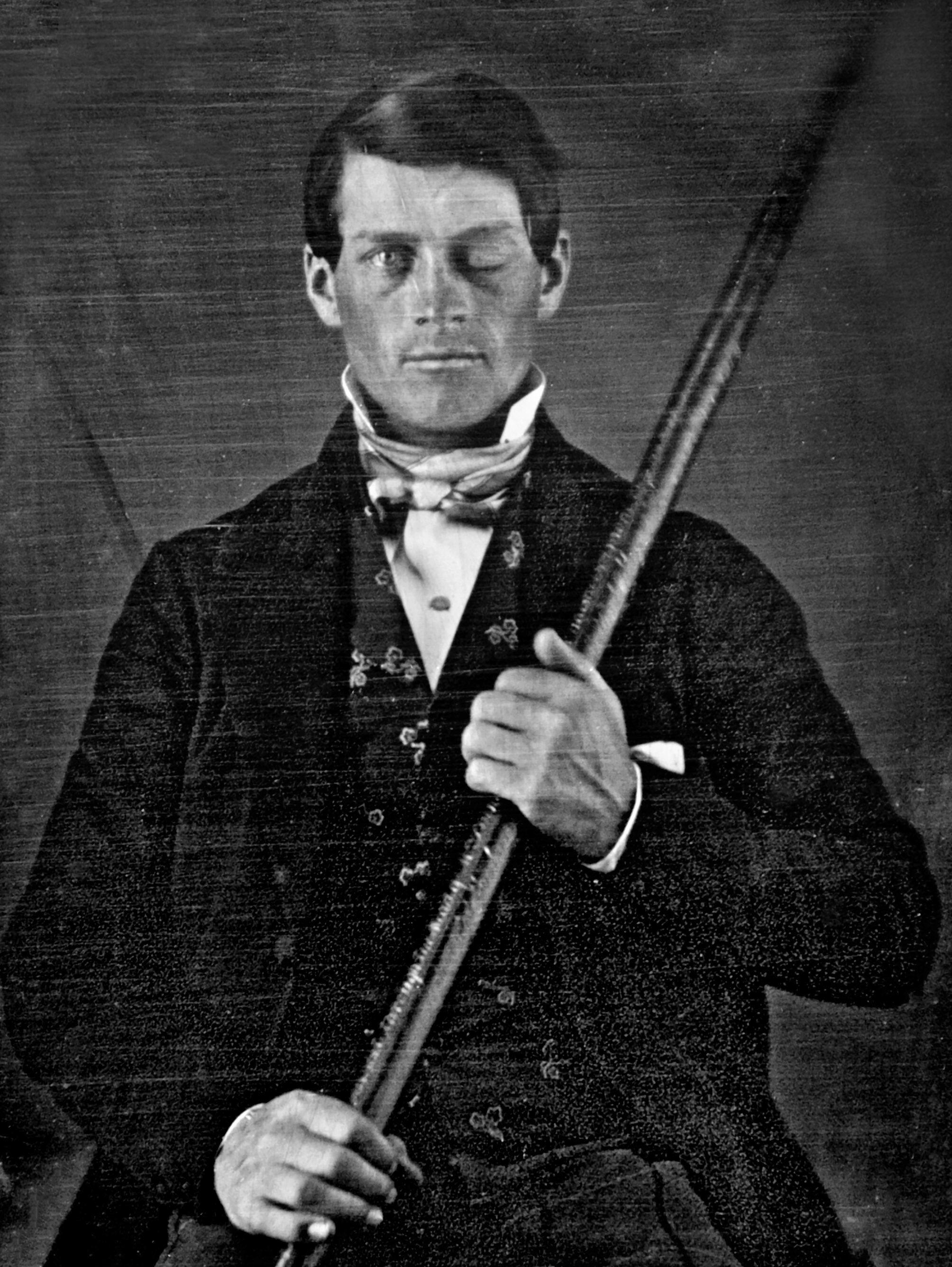
Fortunately, one does not need to have a whole brain region effaced by steel to draw connections between structure and function; modern neuroscience has developed myriad techniques for deriving similar insights from the study of healthy brains. Since the emergence of visualization technologies, such as electron microscopy (EM) and functional MRI (fMRI), scientists have been able to examine healthy brains for data about everything from the movement of water molecules inside axons to the precise location of neural activation when a research subject is asked to do arithmetic in their head. Without trauma, and with the right methods, it seems a healthy brain yields information as richly as a damaged one.
Of the methods used to study healthy brains, connectomics is the most ambitious.
Connectomics seeks to create nothing less than a three-dimensional model of every physical connection between neurons to build a map of the brain. With this map in hand, scientists hope to better understand how neural circuitry dictates and predicts the activity of individual neurons.
However promising an approach, this mapping process currently requires vast amounts of time and resources. In the 1970s and 80s, it took over ten years to map just 302 neurons in C. elegans, a microscopic worm. And even with significant advancements upon 1980s technology, it took researchers over a decade to complete the fruit fly connectome, which possesses only 140,000 neurons, in 2024. Mammalian brains are far larger; a mouse has some 70 million neurons, all intricately studded with axons, dendrites, and microglia. Mapping the mouse connectome is projected to take about 15 years and cost 10 billion dollars, according to a report from the Wellcome Trust. Much of this research is, of course, seen as laying the groundwork for a human connectome, which, at 100 billion neurons (three orders of magnitude more than the mouse), presents a Sisyphean task without the development of new tools to expedite the process.
Fortunately, such tools are already being built. E11 Bio, a Bay area-based non-profit staffed by neuroanatomists and bioengineers, is creating a platform not only to better visualize the neural connections but also to improve the algorithms available to map these circuits. Once these tools are complete, E11 Bio anticipates collaboratively delivering an entire mouse connectome in five years for just 100 million dollars — a 100x improvement over the Wellcome Trust’s estimates — all while open-sourcing their methods.
Should this project succeed, neuroscience stands to benefit not only from the other connectomes that will likely follow but also from the technologies and applications that such structure-to-function insight could give rise to: everything from allowing researchers to devise therapies to restore healthy connectivity in the scores of people suffering from neurological diseases to building an accurate structural representation of the brain for use in brain computer interfaces or whole brain emulation.
{{signup}}
The Dawn of Connectomics
While Phineas Gage might be considered the man who unintentionally launched neuroscience, the true father of the field is the Spanish histologist Santiago Ramón y Cajal. Cajal was born in 1852, and was most productive at the turn of the 20th century, after setting aside chess, pyrotechnics, and other distractions. As a boy, Cajal had dreamt of becoming an artist. And although he dutifully entered medicine at his father’s insistence, his aesthetic bent came through in his work. Using silver nitrate and potassium dichromate to stain slices of brain tissue, Cajal revealed the microanatomy of individual neurons and their connections. He would study these samples all morning, and draw them in the afternoon, often from memory.
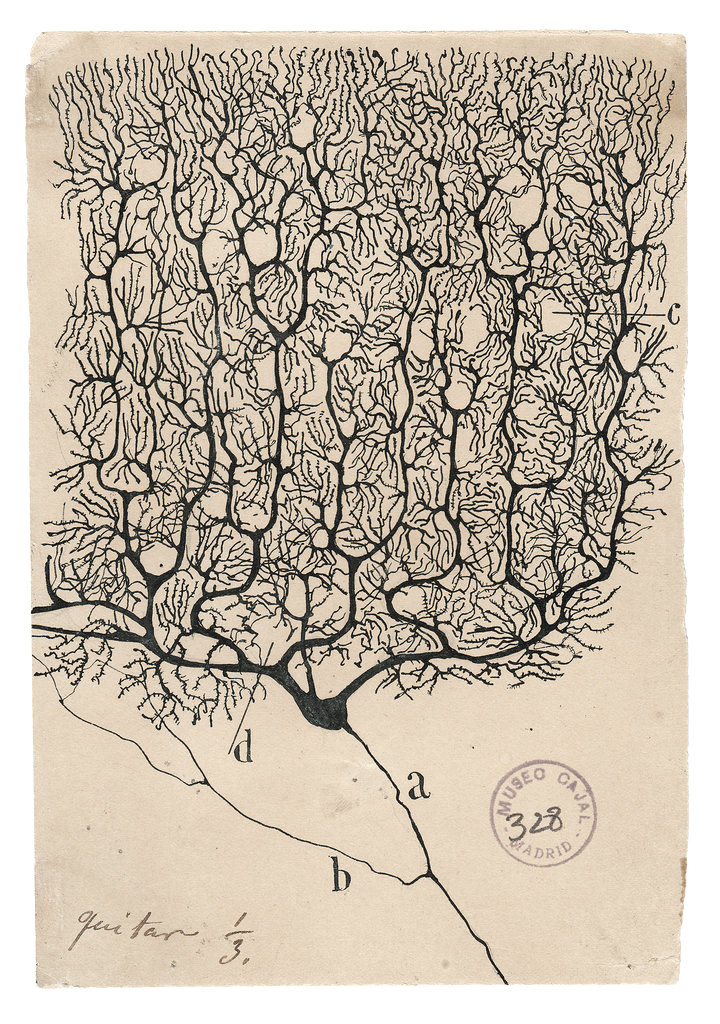
Cajal’s illustrations of brain circuitry are breathtakingly complex, especially considering that he drew them freehand. Even so, they pale in comparison to the intricacy of real synaptic and neuronal connections within our skulls — many of which involve cells finer than the width of a human hair. Still, Cajal worked tirelessly to identify the underlying relationships of these structures, challenging the prevailing wisdom that brains were made from one continuous strand of undifferentiated cellular fibers. Working with the most powerful light microscope available in the late 1800s, Cajal demonstrated that the brain was composed of a variety of discrete cells.
While Cajal’s microscope had enough resolution to detect individual neurons and larger cell types, it could not resolve finer structures like synapses. And though his drawings served to lay the foundations of connectomics, they could provide only static and sparse views of a dense and dynamic structure.
In the decades following Cajal’s observations, technologies emerged capable of revealing the brain’s density and dynamism. The most important of these was the electron microscope. Whereas Cajal’s light microscope could resolve parts of cells as small as 1 micron, electron microscopes could resolve objects at ~0.1–0.0001 microns (a more than 10x improvement). The first synapse was visualized using electron microscopy in the mid–1950s, although parallel work and delicate egos make it difficult to determine with certainty the first researcher to actually see one.1
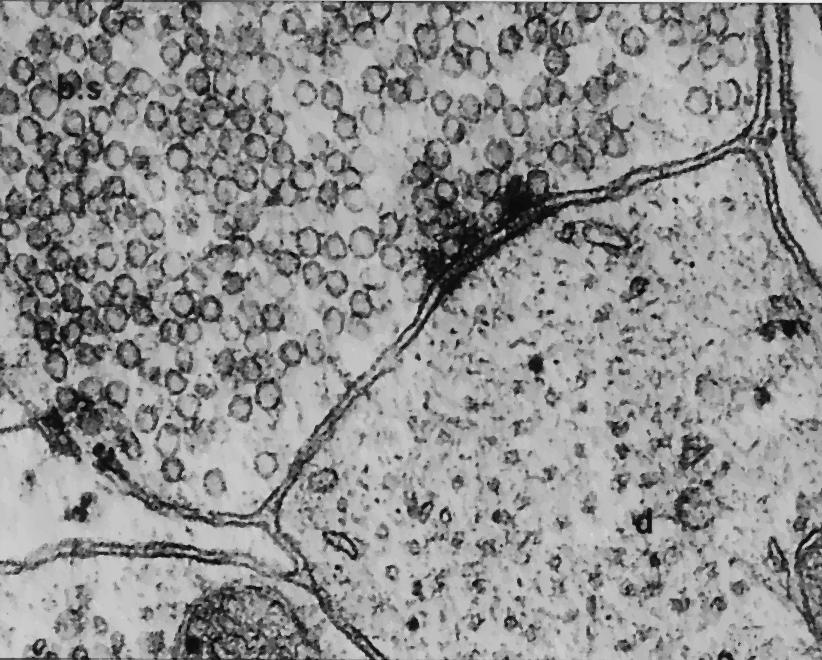
By the 1970s, however, focus had moved from merely visualizing synapses to making sense of them. Confident that structure often determines function, biologists turned their attention to investigating the composition of the brain. The most prolific of these was the South African biologist, Sydney Brenner. For over 20 years, Brenner and his team used electron microscopy to analyze and reconstruct the circuits of the microscopic roundworm C. elegans. They published their results in the journal Nature in 1986, not only presenting “connectomes that encompass the entire animal for the nervous systems of the two adult sexes of the nematode C. elegans” but also asserting the greater promise of connectomes for future neurological and behavioral research:
"To understand animal behaviour, it is necessary not only to identify the cellular substrates and circuits that underlie particular responses and actions, but also to describe how these circuits are integrated to generate a cohesive and prioritized adaptive output. A connectivity diagram that covers the entire nervous system is necessary to investigate how such integration is implemented."
In other words, the promise of connectomics is nothing less than understanding the holistic behavior of an animal — its activity and perhaps even the content of its conscious mind — by tracing its neural circuitry.
Naturally, interest in more complex organisms followed Brenner’s work, and by the 2000s "connectomics" had established itself as a distinct field. In 2006, The Howard Hughes Medical Institute's Janelia Research Campus initiated FlyEM, a project aiming to reconstruct the complete connectome of the Drosophila brain using state-of-the-art EM and computational tools. In 2010, grants from the NIH helped launch the Human Connectome Project (HCP), a five-year effort aimed at mapping the structural and functional connectivity of the human brain using non-invasive techniques such as diffusion MRI (dMRI) and functional MRI (fMRI). It’s worth noting, however, that this effort focused on uncovering macro connections between brain regions rather than micro-connections between individual neurons.
After more than a decade of investigation, researchers unveiled the Drosophila connectome in 2024. At a cost exceeding 40 million dollars, over 140,000 neurons, joined by more than 490 feet of wiring, were offered up for scientific scrutiny.
Work on the human connectome presses on. During the five years that it existed, the Human Connectome Project hit many of its targets. It not only spurred advancements in neuroimaging tools and methods but produced a freely accessible collection of more than 27 Petabytes of data, with 1,538 papers directly crediting its use. But despite such strides into data acquisition, analysis, and sharing, we are nowhere close to a full human connectome able to reveal detail at the level of individual connections.2
Mapping the mouse connectome seems a far more tractable endeavor, and the Wellcome Trust's recent report provides a clear roadmap for achieving this goal. The report suggests that today’s cutting-edge technology will make it possible to deliver a whole mouse connectome in 10-15 years, though the exact costs and timeline remain uncertain. The authors are certain, however, that given the complexity and scale of the mammalian brain over that of C. elegans and Drosophila, “the road to a mouse connectome is a long one.” They elaborate:
[The mouse brain is] over 10,000 times longer than the fly based on the amount of neural wiring in each brain. The mouse brain is a tenth of a teaspoon (500 cubic millimetres) in volume, but this is 500 times what has ever been imaged by electron microscopy; the raw 3D image data for just one mouse brain would fill ~500,000 laptops or the storage servers at the Wellcome Genome Campus.
For connectomics to achieve this monumental task, scientists, technologists, and funders must galvanize around producing the tools necessary to make both the collection and reconstruction of such a large quantity of data manageable. And not only that but at an affordable cost in a reasonable timeframe.
The first step is to target the biggest bottleneck in connectomics so far; namely, electron microscopy.
Modern EM methods are both costly and onerous. Not only do the microscopes themselves run on the order of $1 million each, but they require expensive servicing, trained staff, and special infrastructure, including vibration-free, temperature-controlled rooms with low electromagnetic interference. Modifying or building facilities that can accommodate these needs can easily require $100,000–$1 million. Then, there is the issue of scale, as mapping the mouse connectome would require about 20 scanning electron microscopes — each costing several millions of dollars — operating simultaneously, around the clock, to achieve the necessary imaging rate of 3.2 GHz (a billion voxels per second) to complete the project in five years.
Mapping circuits with electron microscopy is also incredibly laborious and exacting. Firstly, researchers must physically slice brain tissue because electrons don’t penetrate far into the sample. This requires sub-wafer-thin layers: taking individual pictures and stitching them together to render a full, three-dimensional image. The resultant images — black and white and just a single electron in width — prove challenging to read. At such resolution, the cells are clear, but the axons and dendrites remain minuscule filaments, too delicate to detect.
To create a full map of the brain, researchers must superimpose these samples in what is essentially a game of connect the dots. For each layer, they must reconstruct the cell’s 3D geometry, figuring out how all the nubs fit together across the entire series of images. This introduces another problem when the layers get thick enough; by the time one has assembled a multiple-millimeter cube, it becomes difficult to keep track of the samples’ orientation.
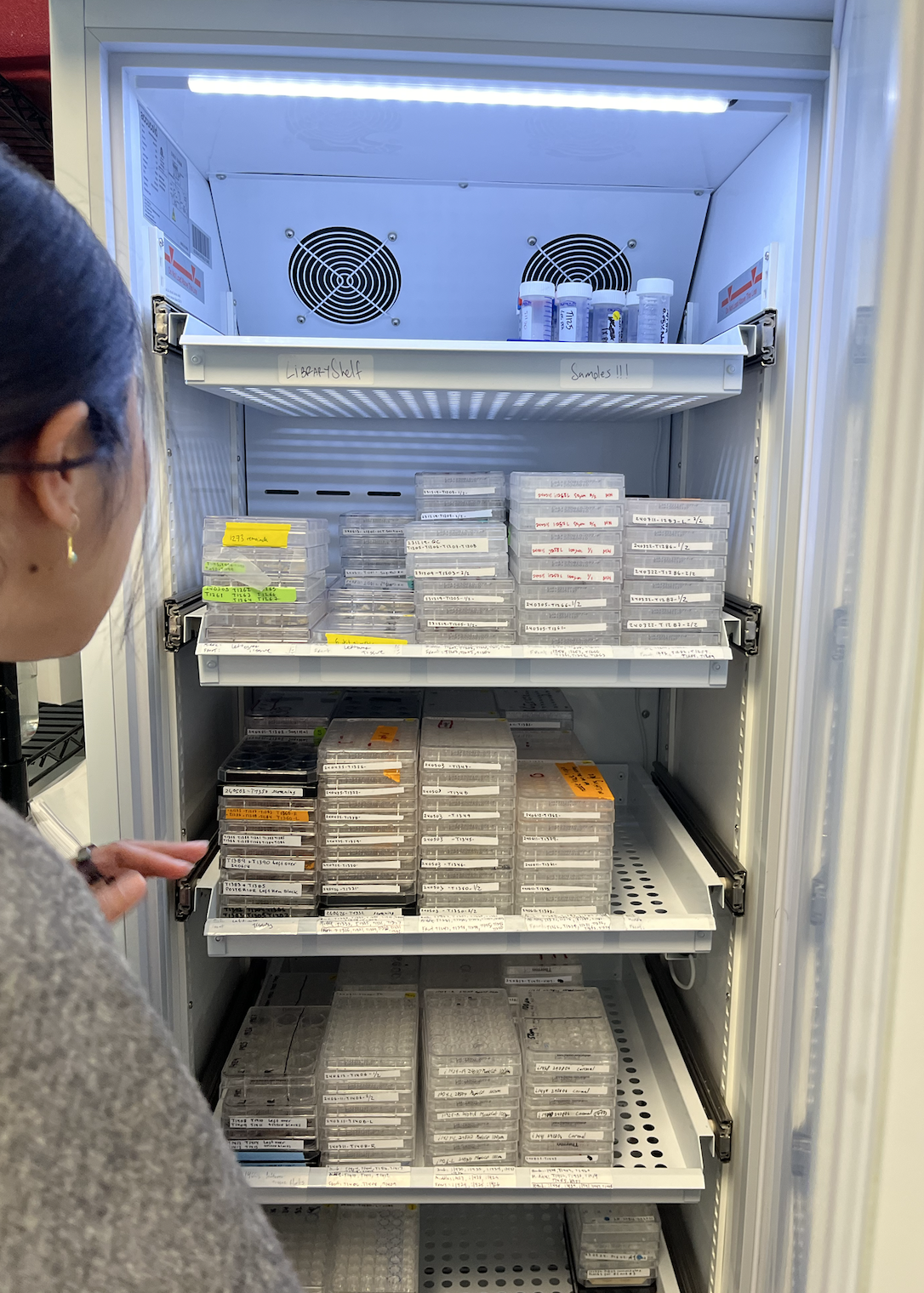
Cutting the brain tissue being imaged presents additional challenges. If one cuts the tissue with a razor, some cells are ablated or damaged where the cut occurred. Any loss of thickness makes it even harder to accurately connect the dots.
Modern researchers rely on algorithms for help. Google Brain, for instance, has developed some of the most advanced algorithms to stitch together neural images, releasing a human millimeter cube dataset in collaboration with a Harvard lab in May of 2024. And although computational aid expedites the process, it has weaknesses. Axons are so small that even the most advanced machine learning algorithms can produce false merges, where a single axon gets read out as two, or false splits, where the inverse happens. Surprisingly, humans are better than ML at spotting these errors. This is what makes brain mapping enormously expensive; when scientists mapped the fruit fly connectome, about 95 percent of the cost stemmed from human proofreading. Extrapolating such a costly method to mammalian brains would be untenable.
This is where E11 Bio comes in.
Andrew Payne, a biotechnologist who previously worked in Ed Boyden’s lab at MIT studying neural circuit mapping, launched E11 Bio in 2022. E11 Bio aims to mitigate the costly, cumbersome process of imaging and proofreading with a method they call “PRISM.” This approach employs two recent synthetic biology breakthroughs — a technique to better visualize neurons and a system to proofread and categorize them more efficiently.
Seeing Better
The first breakthrough E11 Bio exploits is expansion microscopy. Microscopes have distinct resolution limits, meaning they cannot easily “see” exceptionally small things. Rather than boost the resolution of a microscope, expansion microscopy flips this idea on its head and instead expands the size of a sample.
“Expansion microscopy allows us to see individual cells all the way down to the synapses,” says E11 Bio’s Chief Operating Officer, Jun Axup.
Scientists begin by polymerizing the brain tissue slice — that is, permeating it with gels that hook onto the tissue through their negatively charged backbones. The polymer in this case is sodium acrylate, a substance nearly identical to the absorptive gel in baby diapers. When the sample is rinsed, it strips away lingering lipids and sodium ions and leaves proteins behind. The negatively charged backbones remain, repelling one another in the same way magnets do when their like poles are brought together. This repulsion causes the sample to grow isotonically while maintaining its gross anatomy.3 The result of this chemical swelling is a “ghost of the brain” almost two orders of magnitude larger in size, making the tissue significantly easier to visualize.
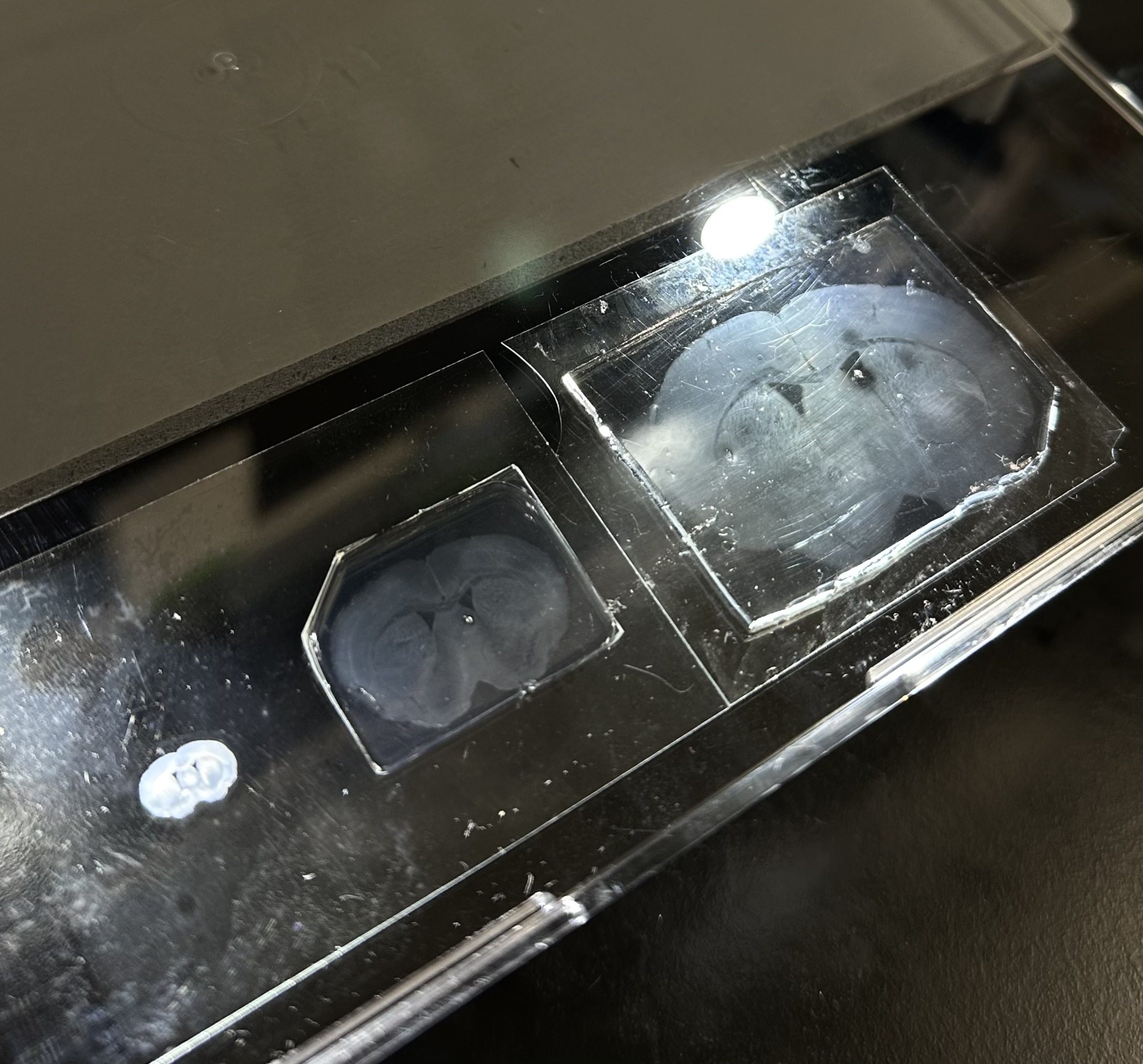
This technique allows researchers to achieve comparable resolution as EM without needing to use it, thereby avoiding the costs and inefficiency it incurs. However, it does not, by itself, solve the proofreading and algorithmic challenges of connectome mapping. That’s where E11 Bio's principal innovation — “barcoding” — comes into play.
Barcoding seeks to improve the current method for mapping brain circuits, which relies on taking a series of pictures and using computer vision to reconstruct those circuits by tracing neuron cross-sections through consecutive images. As evidenced by false splits and merges, this process is far from perfect.
“When we launched the project, we had a thesis that this error-prone step is an enormous bottleneck,” says Andrew. “But we think we can address it by developing protein barcodes that label each cell in the brain.”
Crucially, not just in the brain but by the brain — as the goal is to create protein barcodes as a way to equip the brain with its own proofreading system. E11 Bio refers to this approach as PRISM, short for “Photo-connectomic Reconstruction by Iterative Staining with Molecular Annotations.” Buried in this mouthful is a crystalline and clever idea; to tag each neuron in the brain with its own ID so that images can be more easily stitched together.
To achieve this, E11 Bio synthesizes protein barcodes (short peptides with unique amino acid sequences), packages them into AAV vectors (engineered, non-harmful viruses for drug delivery), and delivers these into the brain tissue. Once in the brain, each cell takes up and expresses a random subset of these tags, so no two cells have the same combination.
Crucially, these cells are not black-and-white as they are when seen in EM, but colored by antibodies that have been linked to a fluorescent dye. When the researchers wash the expanded brain tissue with different sets of these antibodies, each type sticks to its matching protein tag. Then, when examined under a fluorescent microscope, the antibody-tagged brain proteins glow in distinct colors. Because each neuron carries a one-of-a-kind combination of these tags, the resultant pattern of glowing spots forms that neuron’s “ID code.” Instead of having to rely on subtle variations in grayscale, researchers can use these colorful “signatures” to more easily distinguish one neuron from another.

Similar methods have been employed before in circuit mapping. In 2007, a Harvard research team devised a strategy to visualize brain circuits at a coarser resolution by genetically labeling neurons with multiple, distinct colors. Using what they dubbed their “Brainbow transgenes,” they reconstructed hundreds of neighboring axons and multiple synaptic contacts in a small piece of a cerebellar lobe, ending up with an image formed of approximately 90 colors. However, whereas the Harvard team used only four base colors to generate their palette, E11 Bio has developed new techniques to expand to more than 100,000 color combinations using protein epitope tags.
After tagging the neurons and imaging the expanded samples, all that remains is for the self-proofreading AI models to stitch the colors together. While this is not entirely error-free, the software can more confidently draw neuronal boundaries after being fed images that already have a built-in legend — the cellular barcode. The working hypothesis is that this training step will dramatically reduce the labor required to do connectomics although by how much is not yet known.
Why Map?
Connectomics is itself just one facet of neuroscience directed at transforming human health and well-being. In the long term, alongside neurodynamics and computational neuroscience, connectomics could pave the way toward brain-computer interfaces or even whole-brain emulation — rendering an entire brain in silicon rather than flesh. But even in the near term, the impact of connectomics could be catalytic for health and medicine. After all, the complex molecular mechanisms that undergird all of cellular life should become easier to parse apart once we understand how or by how much the connection of these cells contributes to their function.
This is why, according to Payne, “It isn’t just important to understand the brain to satisfy human curiosity but also to find new targets for and develop therapeutics.”
This issue is deeply personal to Payne, who lost two grandparents to neurodegenerative diseases during the course of his PhD. And while not every brain disorder is a connectopathy — that is, a disease relating to the neural connections in the brain — they do comprise an especially deleterious and prevalent subset; including conditions like Alzheimer's disease, wherein a loss of synaptic connections and degeneration of neural networks causes severe cognitive decline, or Schizophrenia, wherein weak fronto-temporal connections and axonal abnormalities are thought to reduce efficient communication between brain regions causing cognitive degradation and delusions. Even mood disorders such as bipolar or behavioral phenomena like autism have been linked to abnormal connections in the brain.
Without more insight into the nature of this circuitry, however, progress in the treatment of these conditions is unlikely to make any great leaps. “Once we have these maps,” Payne says, “you can start changing people's lives by using them.” Yet even despite eminently reasonable speculation such as this, connectomics has its detractors.
"People often ask 'How good is one connectome?',” says Axup. “But, just like the first human genome, it starts as a reference map to help other scientists navigate their neurons of interest. Additionally, there is lots of basic information we don't know about the brain, such as different cell types and where certain neurons project to. However, similar to what we saw with the decreasing cost of sequencing, as the technology becomes cheaper and more robust, we will be able to look at more connectomes and begin to compare them."
Indeed, entire scientific fields have arisen that make use of the rapidity with which we can now sequence genomes (currently possible in less than a day). Metagenomics, for example, allows scientists to build extensive reference libraries of DNA for use in everything from the creation of vital medicines to CRISPR tools.
For a reference connectome to truly unlock comparative connectomics in the same way it did for genomics, it would also have to become cheaper.
However, even if costs surrounding connectomics decrease and it becomes easier, there is considerable uncertainty surrounding how valuable it will actually be in terms of informing scientists about the connection between structure, function, and, ultimately, pathology. As the neurotechnologist Patrick Mineault has commented, brains are complicated and dynamic machines wherein “dendrites and synapses roll over; [and] we can’t always infer the strength of a connection from its static shape.”
The human genome project labored under similar challenges. In a 2000 paper aptly entitled “Finding Function Through Structural Genomics,” researchers Lawrence Shapiro and Tim Harris asked: “Can structural knowledge enable us to efficiently answer early-stage biological questions of protein function?” In what followed, they argued that newly derived three-dimensional structural information would provide insight into the biochemical function of given proteins where two-dimensional sequence information has historically failed. This is why, they suggested, proteins involved in many cancers such as the p53 protein, were overlooked by purely structural studies. Even so, structure carried enough weight to make it “a cornerstone in a strategy for determining protein function.”
So while it would be naive to expect connectomics to tell us everything about the nature of brain disorders, it’s reasonable to expect that structure might reveal a great deal. For example, researchers from HHMI’s Janelia Research Campus and the University of Tübingen used the connectome of a fly brain to model Drosophila’s visual activity. Using detailed maps of neural connections in the fly's visual system, which shows how around 45,000 neurons are wired together across 64 different cell types, the researchers built a computational model that incorporated these exact connections but left certain properties (like how strongly neurons communicate) as adjustable parameters. By optimizing these parameters to perform well on motion detection (by training the models via video) the model naturally developed properties that match what scientists have observed in real fly neurons. They found that they were consistently able to recapitulate the brain activity flies experience when optically navigating flight using only the connectivity information. In other words, electrophysical data, computational models, and circuit data provided insight into function.
Open questions about the extent to which static connectomes can elucidate the behavior of neurons and complex cognitive phenomena do not diminish their importance as a starting point. Just as a city planner looking to tackle traffic wouldn’t only look at moving vehicles but also consider the layout of the roads and intersections, a neuroscientist hoping to understand the life of a mind wouldn’t only look at emergent and dynamic phenomena like consciousness without first considering brain circuitry.
In the event that a connectome did yield insight into function, other downstream technologies would still be necessary to uncover what Cajal referred to as “the secret of mental life.” But where Cajal was interested in understanding how brain structure might relate to modeling health or consciousness, these contemporary technologies go even further: improving health and performance outcomes beyond what is currently possible and even simulating consciousness.
Addressing the former, technologists like Mineault have imagined how neurotech might lead to the development of stronger and more precise neuroprosthetics or the enhancement of perceptual learning. And looking to the latter, researchers have spent decades discussing how a better structural understanding of the brain and electrophysiological models might enable us to digitally simulate human intelligence in the form of whole brain emulation (WBE).
A prescient 2008 report from the Future of Humanities Institute remarked how, even in light of being an as-yet “theoretical technology” rife with open engineering, neuroscience, regulatory, ethical, and social questions, brain emulation was worth roadmapping. Its authors remark:
Our view is that when the stakes are potentially extremely high, it is important to apply the best available methods to try to understand the issue. Even if these methods are relatively weak, it is the best we can do. The alternative would be to turn a blind eye to what could turn out to be a pivotal development. Without first studying the question, how is one to form any well‐grounded view one way or the other as to the feasibility and proximity of a prospect like WBE?
The stakes have risen higher still alongside growing concerns over the possibility of unaligned artificial intelligence. In the event that human intelligence could be uploaded to more safely guide the development of AGI, accelerating the technology that enables this becomes increasingly urgent. Researchers are already finding that models whose features are made more “neural” — that is, regulated by the inclusion of more raw neural data — are less vulnerable to adversarial attacks and random noise. Yet more data (perhaps a whole human brain’s worth), they conjecture, “will further enhance the robustness and generalization performance outside the training set.”4
With similar studies suggesting how neuroscience data can positively influence AI safety, connectomics may have found its directive. Add this to its potential impacts on health and medicine, and the value of connectomics becomes even starker. However, even without conjecture about its future applications, a connectome is a formidable explanatory tool. Cajal once observed that "As long as our brain is a mystery, the universe, the reflection of the structure of the brain, will also be a mystery." It follows that better understanding the exterior world begins, to some extent, inside our own brains. This is consistent with scientific theories for consciousness that posit that conscious experience arises from internal predictions — from inside our brains outwards, rather than the other way around.
As research groups like E11 Bio continue to advance cheaper and more efficient connectomics, fields as disparate as philosophy, AI safety, cognitive science, and biomedicine move ever closer to their ultimate aims. What began with a minuscule roundworm expands, as Cajal hoped, to encompass “the universe.”
{{divider}}
Xander Balwit is editor-in-chief of Asimov Press.
Cite: Balwit X. “Barcoding Brains.” Asimov Press (2025). DOI: 10.62211/16oe-73ht
Reporting for this article was made possible by Convergent Research.
Lead image by Iris Fung.
Footnotes
- In 1955, neuroscientist Stanley Palay published the first EM study of the neuron and the central synapse in vertebrates’ central nervous system while working at the Rockefeller Institute with his colleague George Palade. Concurrently, Eduardo De Robertis and Stanley Bennett published their work on ‘‘Changes in the ‘Synaptic Vesicles’ of the Ventral Acoustic Ganglion After Nerve Section (an Electron Microscope Study).” While there might be some distinction to draw between “discovering” and “fully describing” that awards both teams of scientists the honor, Palay’s position was clear; In his memoir, he (1992, 209) wrote ‘‘I well remember the moment when I discovered synaptic terminals [including vesicles] in my preparations.’’
- For a 2024 paper, however, a team of neuroscientists lead by Jeff Lichtman shared a dataset on the reconstruction of a part of a human temporal cortex, 1 mm3 in volume, which included thousands of neurons, more than a hundred million synaptic connections, and all of the other tissue elements that comprise human brain matter.
- The swelling process does not take long. I could see the sample slowly expanding after only a few 10-minute rinses and repeats.
- There is no consensus among experts on whether WBE will be good for AI safety. While it may be more interpretable than other forms of human-level artificial intelligence (and, therefore, easier to interpret and control), it is still a form of transformative AI, which means it entails a certain amount of risk.
This article was published on February 9, 2025
Always free. No ads. Richly storied.
Always free. No ads. Richly storied.
Always free. No ads. Richly storied.