Driving Toward Nanopores

An organism’s entire genome can be sequenced today using little more than a laptop and a $1,000 device, slightly larger than a smartphone, called a nanopore sequencer. The smallest of these measures just four by one-and-a-half inches across and one inch thick.
Such a device seems implausible. But these little machines, small enough to fit inside a pocket, have become commonplace. Biologists use nanopores for everything from diagnosing diseases and monitoring changes within rainforest ecosystems to discovering proteins from microbes frozen in Icelandic glaciers. And unlike traditional DNA sequencers, which parse genetic material by breaking it up into fragments and interpreting it chunk-by-chunk, a nanopore device unspools a long strand of DNA and reads it all at once.
The inside of each device contains thousands of nanoscopic proteins with holes in their center—a pore—through which molecules pass. As individual nucleotides—A, T, C, G—travel through these pores, the nucleotide briefly blocks the channel and disrupts an electrical current. A delicate sensor, positioned on the other side of the membrane, senses these electrical “squiggles.” And finally, a computational algorithm decodes these data to reconstruct a DNA sequence.
Nanopore devices work incredibly fast. A scientist can isolate DNA and load up a flow cell in fifteen minutes. Hospitals harness this speed to sequence and analyze tumor DNA within 6 hours, more than twice as fast as similar analyses performed using traditional next-generation sequencing, such as Illumina. The devices also read long strands of DNA continuously, making them particularly well-suited to applications where re-assembling a genome’s contents from shorter fragments is difficult. Not long ago, a nanopore device “filled in” the remaining 8 percent of the human genome, a portion once thought to be unsequenceable because it contains lots of repetition that traditional sequencers could not decode.
Finally, nanopore sequencers are portable. Scientists recently trekked through the Ecuadorian rainforest with little more than a backpack and nanopore device, using it to rediscover many rainforest species, including a Jambato toad (Atelopus ignescens) thought to be extinct for 28 years.
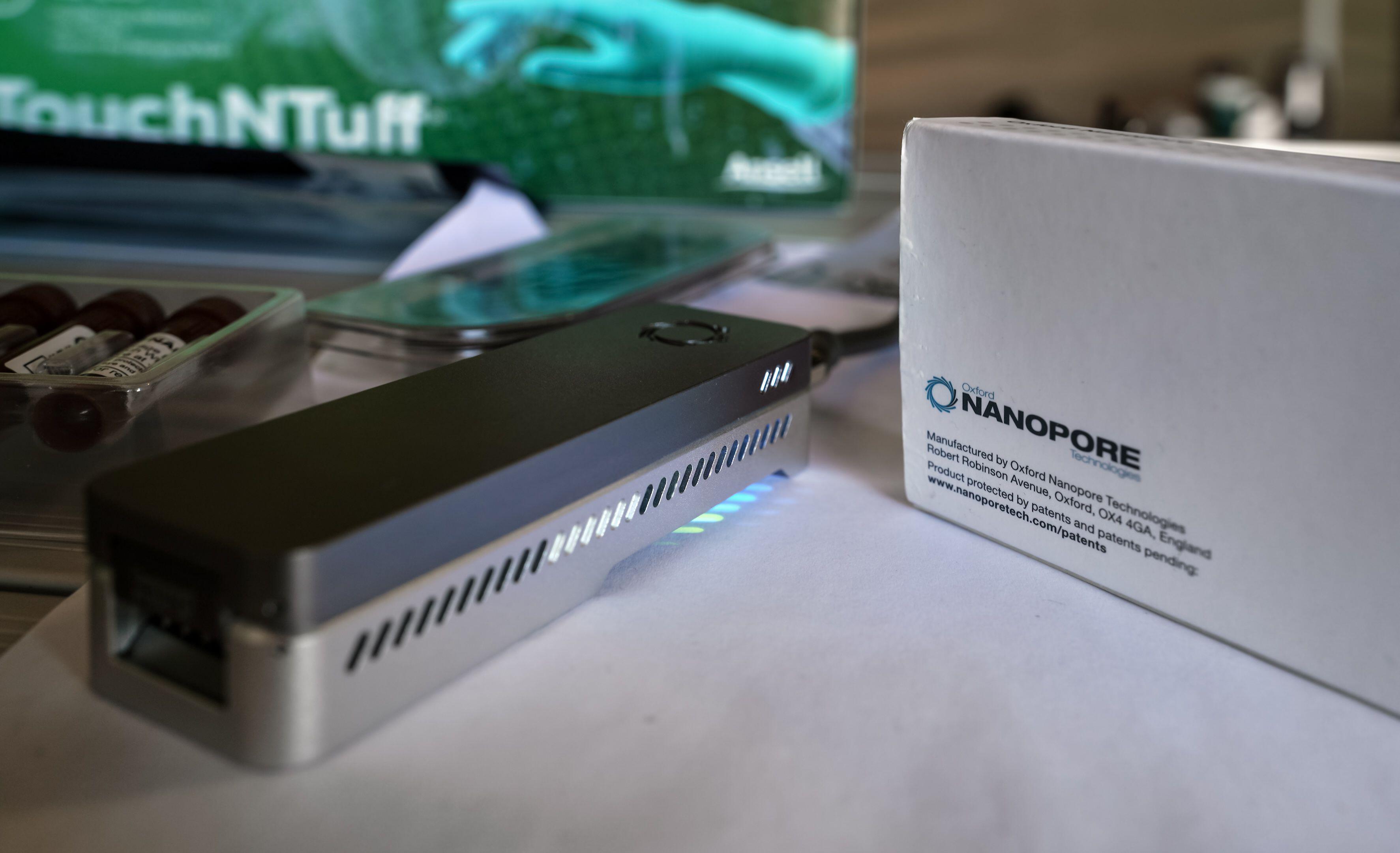
Progress in nanopores holds lessons for other measurement technologies. Any device—be it a ruler, microscope, or DNA sequencer—needs to be sufficiently cheap, simple, and accurate before it can be widely adopted. Just thirty years ago, nanopore devices were none of these things. Costs and ease-of-use improved as scientists graduated from working on delicate prototypes to working at companies that manufactured devices by the thousands. Solving the accuracy problem, however, took a bit longer.
Before the integration of machine learning algorithms, early nanopore devices were often unable to distinguish between individual nucleotides. Today, machine learning is so tightly integrated into nanopore sequencers that some devices bundle onboard GPUs to speed up model inference.
This is the story of how disparate inventors came together to turn an idea that began on a scrap of notebook paper into a physical measurement device that has become ubiquitous.
{{signup}}
Dreams While Driving
In 1989, an American biologist named David Deamer was driving along a California highway when he had a strange vision, perhaps induced by the starts and stops of endless traffic. Deamer imagined a strand of DNA threading its way through a tiny protein channel that could “magically” decode its sequence.1 At the time, Deamer was a professor at UC Santa Cruz, where he’d moved to join his wife, a professor of biochemistry. Deamer had been studying rudimentary artificial cells and was interested in how biological systems move molecules across cell membranes.
Behind the wheel, Deamer realized that because different nucleotides in DNA all have a unique molecular shape, this might be used to distinguish them as they passed through a narrow channel. The channel could be charged with an ionic current, Deamer mused. As each nucleotide passes through, they would disrupt the current and leave behind a tell-tale fingerprint on the electrical signal.
It took Deamer a full seven years to produce a proof-of-concept of his nanopore “dream.” In 1996, he demonstrated that a nanopore built from ɑ-hemolysin, a pore protein derived from Staphylococcus aureus bacteria, could discriminate between long and short strands of DNA. Deamer chose this particular protein after reading papers that indicated that the ɑ-hemolysin protein has an internal diameter of 1-2 nanometers and was therefore large enough for nucleotides to pass through.
But ɑ-hemolysin had a flaw; namely, a frustrating tendency to open and close repeatedly, preventing objects from flowing smoothly through its channel.
In 1992, physicists Sergey Bezrukov and John Kasianowicz figured out a way to keep ɑ-hemolysin channels open by embedding the protein in a bilipid membrane and flooding it with a potassium salt solution. A negative electrode was placed on one side of the protein—near the DNA to be sequenced—and a positive electrode was placed on the other side of the membrane. The voltage difference between the two sides pulled the negatively charged DNA toward the other side, forcing it to thread through the protein pore. The DNA molecules generated tiny perturbations to the electrical current as they flowed through.
This initial nanopore prototype was unable to distinguish between individual bases but did demonstrate that nucleotides could enter and exit through a protein channel: a feat that other scientists had speculated would be the biggest hindrance in building a functional nanopore sequencer. This 1992 experiment suggested, for the first time, that nanopores could be viable.
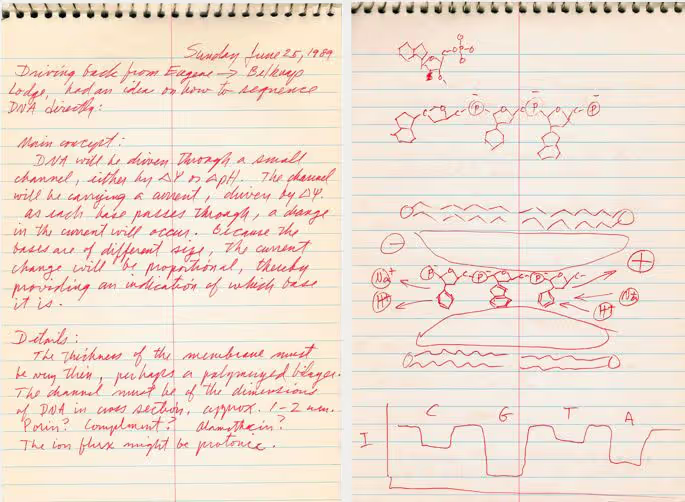
But here, Deamer and his collaborators ran into a problem: speed. The nucleotides passed through the pore so quickly—about one base every 1-10 microseconds, or 1,000 times faster than a flap of a bee’s wings—that it was difficult to detect individual nucleotides as they flew by.
A partial solution to the problem came in 2005 when a team of researchers at the Scripps Institute found a “hacky” way to slow down the speed of nucleotides. Their solution was to fuse hairpins, little loops made from RNA or DNA, at various positions along the DNA strand that was being sequenced. As the DNA passed through a nanopore, these hairpins would get stuck inside the nanopore and slow down the DNA’s movement.
For one experiment, the researchers attached hairpins to a DNA strand that contained only Cs and As. As the DNA strand moved into the nanopore, the hairpins scraped against the channel’s walls and slowed it down. At one point, the DNA became completely stuck within the pore, resulting in a long, sustained disruption to the electrical current. This experiment demonstrated that one could lengthen the time the current was blocked by passing nucleotides slowly through a channel, and then use the data to identify individual nucleotides in DNA.
In the years that followed, nanopores gradually continued to improve. The largest advances came from two factors: better nanopore proteins and a more consistent way to slow down the DNA strands passing through them.
While the ɑ-hemolysin protein from Staphylococcus was good enough for early prototypes, the protein’s “sensing region”—the part of the protein pore that becomes blocked and actually disrupts the electrical current—was too large. A full twelve bases of DNA fit inside of the protein at once, which made it exceedingly difficult to detect individual bases.
But in 2008, physicists at the University of Washington made a nanopore sequencer using a protein, called MspA, from Mycobacterium smegmatis, a bacterium first described in the 1880s. The bacterium uses this particular protein to take up nutrients from its environment, but serendipitously the protein also possessed just the right pore-sensing region shape and span—narrow and short—to sharpen the fidelity of the electrical signal.2
Scientists next went searching for an easier way to slow down the translocation of DNA by a factor of 10 to 100, thus making it simpler to distinguish individual nucleotides as they passed through a nanopore. In 2012, they found it in the form of DNA polymerase, an enzyme that replicates the genome prior to cell division. DNA polymerase latches onto DNA and makes an identical copy to pass down to a cell’s progeny. And it does so at a leisurely pace, at least by biology’s standards: about 200 nucleotides per second.3
Nanopore engineers decided to cleverly exploit the natural speed limit of DNA polymerase to slow down DNA translocation through the pores. Their key insight was to use DNA polymerase’s copying process to “ratchet” DNA through the channel one base at a time. To accomplish this, they mixed together a bacteriophage’s polymerase enzyme with a DNA strand. The DNA was also modified to ensure that the polymerase could attach to it, but would remain inactive until the molecules were captured by the nanopore. Once that happened, the polymerase ratcheted the DNA through the nanopore at a slow, steady rate.
This experiment reduced signal blur in the electrical currents and set the stage for nanopore sequencing with single-base resolution. Now equipped with more serviceable proteins and clever methods to slow down DNA traffic, nanopores could finally evolve from a niche science project to an industrial sequencing workhorse.
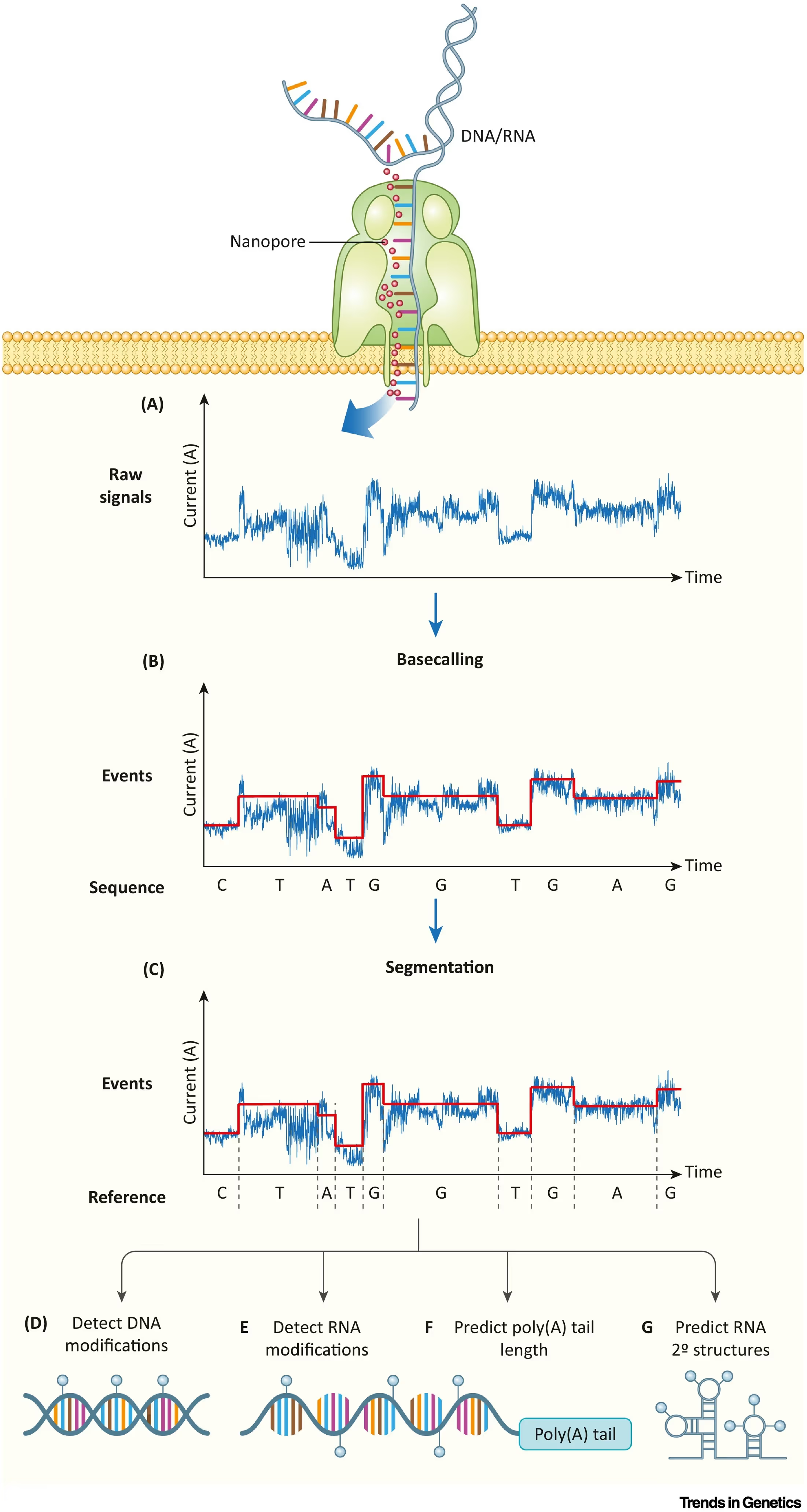
Accurate and Affordable
Like any measurement device, the nanopore needed to become accurate, cheap, and easy to use before it could reach widespread adoption. Academic labs had made a working proof-of-concept as early as 1996, but by the early 2000s, it became clear that a company would be needed to support the sustained investment required to make nanopore devices at scale.
Hagan Bayley—a member of the Scripps team that used hairpins, in 2005, to slow down DNA passing through a nanopore—founded a company called Oxford Nanopore, alongside Gordon Sanghera and Spike Willcocks, that same year. The trio released the first nanopore-based sequencing device, the minION, in 2014.
Over the last decade, the company has made important improvements to the technology, including reducing its cost and boosting its speed. It now takes just a few minutes to “load” the device with DNA and costs anywhere from $21 to $425 to sequence one billion bases. That price point is comparable to Illumina machines, at between $50 to $63 per billion bases. Modern nanopore devices can also detect epigenetic marks, such as methylation groups, attached to DNA—something that Illumina cannot do.
When Oxford Nanopore released the minION, it immediately stood out for its long read lengths but, less auspiciously, for its lackluster accuracy. The device correctly identified each base as it passed through a pore only 70-80 percent of the time. Meanwhile, Illumina’s machines achieved upwards of 99.9 percent accuracy. This large gap limited the nanopore’s use, especially for applications that required high accuracy, such as in clinical diagnoses.
Closing this chasm in accuracy would hinge, in large part, on algorithmic improvements to the DNA base calling step. A base caller is the algorithm that predicts the identity of each nucleotide passing through a nanopore by decoding the raw, electrical signal waveforms (the “squiggles”). Even after technological refinements were made to the pore proteins and the speed at which DNA passed through them, accurately deciphering these “squiggles” remained difficult. Small molecules can randomly transit through the pore and create disruptions, and the polymerase ratchet mechanism is imperfect, occasionally causing DNA to temporarily reverse direction through the pore.
More fundamentally, though, the electrical current “squiggles” are determined not only from individual bases but also from their neighboring contexts. In other words, the electrical signal generated from an ‘A’ will look different if that ‘A’ is surrounded by Cs or Ts. Base callers were therefore developed to decipher k-mers, or short sequences of DNA, rather than individual bases to skirt around this problem.
In 2015, Oxford Nanopore spun off another company, Metrichor, to provide base-calling algorithms through a web interface. Metrichor’s base caller used a hidden Markov model (HMM), a probabilistic machine learning algorithm, to decode DNA sequences. But few details about how this model works were ever released. Fortunately, just two years later, academic computer scientists in Toronto released an open-sourced base caller, called Nanocall, that also relied on an HMM to decipher fluctuations in a nanopore’s electrical current.
Hidden Markov models represent probabilities of transitioning between different k-mers, but they only consider two data points: The immediate signal and the k-mer that passed through the pore just prior. Simple statistical models then relate the “squiggle” to the k-mer. However, because these models only considered a tiny window of data, they were unable to factor in broader surrounding contexts that might improve base-calling accuracy.
To solve this problem, base callers began to be built from recurrent neural networks (RNNs), which model entire sequences. These sequences can consist of words, audio, or, in the case of nanopores, “squiggles” in an electrical current. Recurrent neural networks keep track of important information about sequences in an internal “memory” that allows them to use information from larger windows of data to make accurate predictions. Relative to HMMs, this means RNNs’ base calling predictions factor in information about surrounding nucleotides in the strand, and not just the few nucleotides situated within the pore at any given time.
The shift to deep learning methods, such as RNNs, unlocked dramatic gains in sequencing accuracy. One carefully designed comparison suggested that the initial shift to deep learning methods for base callers catalyzed anywhere from a 2 to 11 percent jump in raw read accuracy for nanopore sequencers.
Data from Oxford Nanopore suggests that today’s nanopores can achieve a raw read accuracy of more than 95 percent, meaning that tailored machine learning and protein engineering efforts added a full 20 percentage points in accuracy in just one decade. However, because the company retrains their base calling algorithms with each new pore release, it’s difficult to disentangle the individual impact of algorithms, data, and pore improvements that made nanopores such a powerful tool for biology.
Making Measurements
Echoing other innovations, the creation of the nanopore involved many actors and contingencies. Deamer dreamt up the initial concept, Oxford Nanopore figured out how to reduce the costs and make the devices easy to use, and computational biologists at Metrichor devised algorithms that boosted base-calling accuracy.
The story of the nanopore bears many resemblances to that of micropipettes, the hand-held tools that scientists use to measure liquids and transfer them from one tube to another. The first commercially-adopted micropipette was invented by Heinrich Schnitger in 1957, but his invention did not reach widespread use until the mid-1970s, when people like Warren Gilson in Wisconsin and Kenneth Rainin in California set up companies to refine and manufacture the devices at scale.4
But whereas a micropipette deals with microliter volumes—consisting of billions of molecules—a nanopore must accurately measure individual molecules. Hardware improvements were good enough to make micropipettes accurate, but hardware alone wasn’t enough to push nanopores from hand-made prototypes to a highly accurate and commercially-available product. Deep learning methods were also required.
Even though the nanopore’s machine learning methods are specialized, how these devices came to be offers important lessons for other measurement technologies. Today, much of biology relies upon precise measurements. Gene expression data can be captured with high resolutions across both space and time, and scientists can create stunning videos of living cells or take point-in-time snapshots of DNA, RNA, and proteins in tissues.
Once these data are collected, though, algorithms must often be used to “clean up” the mess. Biology, after all, is quite noisy; scientists must contend with contamination, nebulous results, and unpredictable disturbances. Taking nanopore’s lessons to heart suggests that machine learning, tightly integrated and improved in lockstep with the refinement of a measurement tool’s hardware, can result in dramatic accuracy gains. It also offers hope that, by doing so, we can decode even the thorniest complexities of biology.
{{divider}}
Stephen Malina is Head of Engineering at Dyno Therapeutics, a company using machine learning to design better viral vectors for gene therapy. More of his writing can be found at stephenmalina.com and an1lam.substack.com.
Cite: Stephen Malina. “Driving Toward Nanopores.” Asimov Press (2024). DOI: doi.org/10.62211/61er-04nb
Footnotes
- This wasn’t the first time that hugely consequential inspiration struck a biologist while driving; the same thing happened to Kary Mullis, a key developer behind the modern polymerase chain reaction method, as he was wending his way through Mendocino County in 1983.
- The natural form of MspA has a negatively charged amino acid within its channel, however, which prevented negatively charged DNA from passing through. This problem was easily resolved by engineering a version of MspA without that particular amino acid.
- This speed varies depending on the organism. A value of 200 nucleotides per second is typical for certain bacteriophages, viruses that infect bacteria.
- Joseph Lister, the British surgeon who pioneered methods to reduce infections during surgeries, also made a micropipette as early as 1857. But the design—which used a long, glass needle to suck up liquids—did not catch on.
This article was published on June 9, 2024.
Always free. No ads. Richly storied.
Always free. No ads. Richly storied.
Always free. No ads. Richly storied.