On Prions and Protein Design

Insights into biology are often uncovered in the study of tiny things. And much of what we consider dogma today emerged from the exploration of specific microorganisms.
Experiments performed with the bacterium Streptococcus pneumoniae not only led to the discovery of DNA as the principal hereditary molecule but also yielded early tools for genetic engineering. The study of RNA tumor viruses revealed reverse transcriptase, an enzyme integral in studying RNA biology. These model microorganisms have long served as natural laboratories, allowing the decoding of life's fundamental principles while providing the very tools needed to study them.
In moving from microorganisms to macromolecules, biology has now entered its protein era. Recent advances in artificial intelligence, including tools like the Nobel-winning AlphaFold, have made it possible to predict protein structures, transforming a painstaking laboratory process into a computational task that can be completed in minutes.
Armed with such insight, biologists hope to design enzymes that could catalyze entirely new reactions, like turning plastic into usable fuel or making new medicines via custom-built catalysts. However, despite rapid progress in the field of protein biology, “core” features of these molecular machines — how they fold, how they change shape, and how simple strings of amino acids can yield forms of incredible complexity — remain unknown.
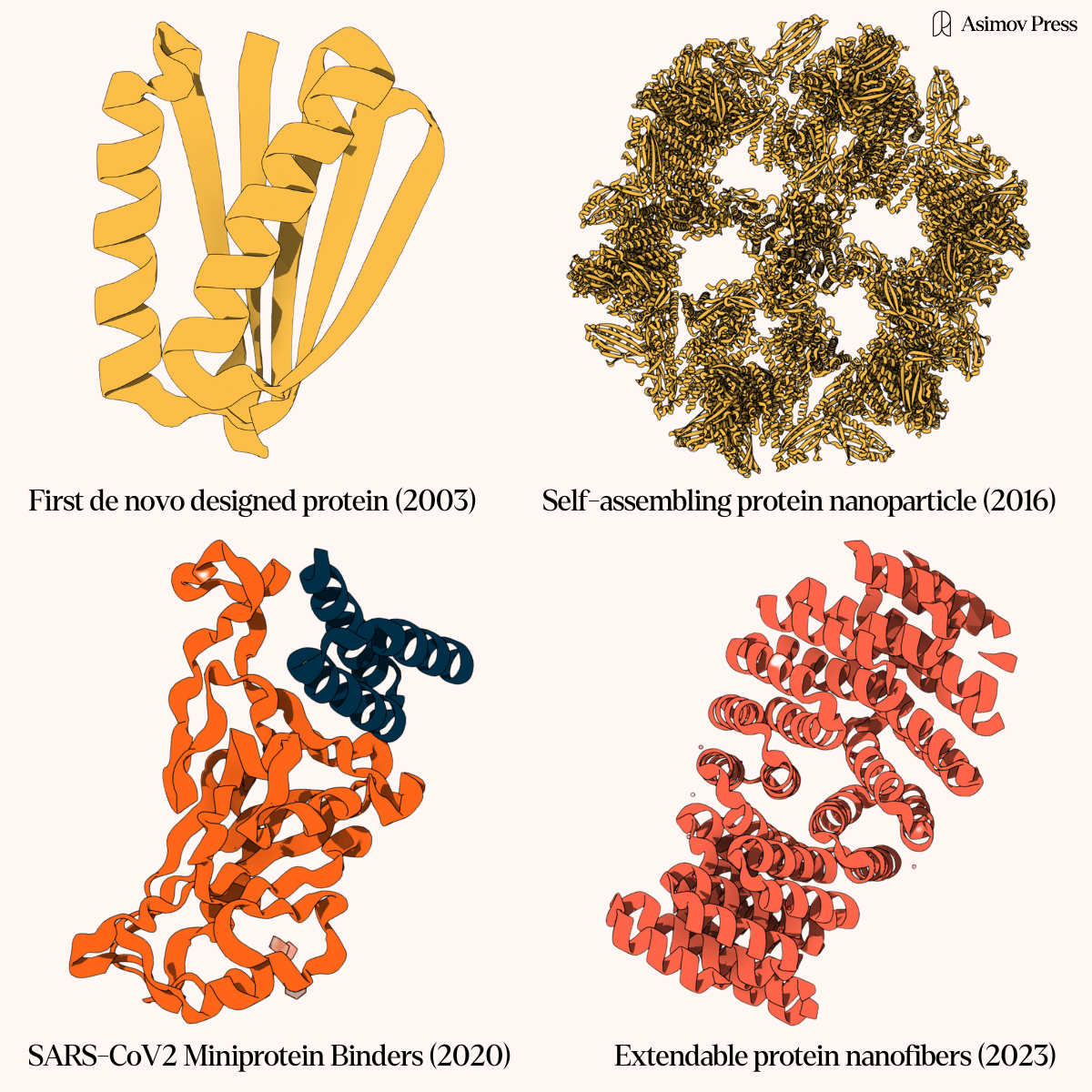
Historically, biologists have investigated protein structure through crystallography and electron microscopy, methods that capture static snapshots of molecules frozen in time. While this approach has yielded many insights, these tools are far too slow to capture protein dynamics. There is, however, another approach that may help: the systematic study of specialized proteins like prions — proteins with uniquely mutable conformations that drive infectious and other behavior. Learning to use prions as nature’s laboratory for modeling protein dynamics may move us closer to mastering protein design as a whole.
{{signup}}
An Infectious Idea
Most people have at least a rudimentary understanding of how viruses and bacteria operate, but prions remain enigmatic. Even their most notorious manifestation, Mad Cow Disease, seems a cautionary tale about contaminated beef rather than a window into one of biology's most fascinating mechanisms.
Prions are proteins that can exist in at least two different shapes, or conformations. In their normal form, they carry out useful functions in the cell, such as signaling. In their abnormal shape, however, they gain the ability to convert other copies of the same protein from their normal state into the misfolded one — a process known as template-mediated conversion. This conversion happens through direct contact between proteins.
When a misfolded prion protein (often denoted as PrPSc) meets its normally-folded counterpart (PrPC), it acts as a template, forcing the normal protein to reshape itself. Each of these can then convert others, creating an exponential cascade similar to a molecular domino effect. This happens because the misfolding exposes hidden, sticky regions of the protein, driving them to clump together into larger structures called amyloid fibrils. These fibrils disrupt normal cellular function, producing irreversible diseased states. Crucially, this process requires neither DNA nor RNA; proteins alone influence other proteins to change shape.
Scientists once considered such behavior impossible. Yet in 1982, after ten years of research, Stanley Prusiner and his San Francisco research team made a revolutionary discovery while studying sheep infected with scrapie, a fatal neurological disease. From diseased brain tissue, they isolated an infectious agent unlike anything known before. It contained no DNA, no RNA, and no viruses — just a single protein. Prusiner dubbed the protein a “prion,” short for “proteinaceous infectious particle.” By demonstrating that proteins alone could spread disease, his work shattered fundamental assumptions about disease transmission.
Prusiner’s work on prions was initially met with skepticism and even ridicule from eminent scientists, including Nobel Laureate David Baltimore. His group’s submitted Science paper sat in a post-revision limbo for months out of fear of the implications of his results.

“It is difficult to convey the level of animosity that both the word ‘prion’ and the prion concept engendered,” Prusiner later wrote of his discovery. After all, how could a protein replicate without DNA or RNA? But just as other paradigm-shifting ideas before it, his “heresy” would prove revolutionary. Through a series of meticulous experiments during the 1980s and 1990s, Prusiner outlined the mechanism behind prions’ destructive cascade. While this work established prions as the causative agent in devastating conditions like Creutzfeldt-Jakob disease and kuru, important questions remained about whether these self-replicating proteins could be more than agents of disease.
Other researchers, such as MIT’s Susan Lindquist, elaborated on Prusiner’s discovery by studying prions in new contexts. For much of her career, Lindquist focused on yeast prions and their inheritance patterns. In 1994, for example, she revealed that a self-propagating protein structure — Prusiner’s prions — could serve as epigenetic elements, passing information between generations without changing DNA sequences. Drawing direct parallels between neurodegenerative diseases and the unusual inheritance patterns she observed in yeast, Lindquist said: “They are all experiencing the consequences of misfolded proteins.”
Reed Wickner's landmark discovery of beneficial prions in yeast further expanded their significance beyond pathology, suggesting that prion-like behavior might be a fundamental mechanism of protein-based inheritance, where an enzyme becomes active only after its inactive precursor is converted into an active state.
More recently, researchers at Columbia University uncovered an even more intriguing role for prion-like proteins: They appear to encode for long-term memories. Proteins like CPEB in sea slugs — which normally bind to RNA and tune protein synthesis — and CPEB3 in mammals convert from their soluble form into aggregated states, triggering the protein synthesis necessary to maintain those memories. This discovery suggests that prions might be a fundamental way that cells maintain lasting changes rather than purely a disease mechanism.
Of particular interest is how prions’ extreme stability flouts common wisdom about protein folding. Most functional proteins exist in a delicate balance between fixity and flexibility — sturdy enough to maintain their shape but flexible enough to do their job. Prions do both; they undergo big conformational changes that require significant molecular mutability, but once converted, they also form robust aggregates that resist almost all attempts at denaturation.
While simple sterilization solutions like ethanol or bleach easily disrupt bacteria and viruses, in their misfolded state, prions’ fixed aggregates are extremely hard to perturb. None of the laboratory’s typically reliable mechanisms of disposal and denaturation — including autoclaving up to 600°C, ultraviolet light, bleach, ethanol, and mild acid — effectively neutralize prion-infected surfaces and disable their infectious states.
This stability is thought to involve what's called "cross-β structure" — a particular way that proteins stack together into extremely stable sheets. This same structural motif appears in diseases like Alzheimer's and Parkinson's, where proteins also form immutable aggregates called amyloid fibrils.
Their ability to change from a flexible to near-crystalline state makes prions uniquely valuable for studying both extremes of the protein stability spectrum, helping us design better therapeutic antibodies that remain stable during storage but are flexible enough to bind their targets or create molecular switches that change state on demand. Understanding their resilience could also be key to engineering proteins to perform in more extreme environments, such as deep-sea vents or industrial reactors functional in deep space.
Even more importantly, prions, with their well-defined transitions between states, could provide the perfect training data for teaching AIs to predict protein dynamics.
The Structural Revolution
For most of modern biology's history, solving a protein's structure involved herculean effort. X-ray crystallography has been the primary tool for structure determination since Max Perutz and John Kendrew first revealed that of hemoglobin in 1960. The process shoots X-rays through proteins and uses the resulting diffraction patterns to reconstruct their 3D structure. However, this requires coaxing proteins into crystals — a process that might take years of painstaking optimization if it worked at all.
In one of the most extreme displays of X-ray crystallography’s finicky nature, one crystallographer dryly noted that "Sweet & Snappy Classic brand pickle juice was found to be the only condition that successfully crystallized our mutant protein." Such an anecdote highlights just how much of protein crystallography remained more improvisation than science, with success often depending on a willingness to try outlandish experimental methods.
The ability to understand protein structure improved with the development of cryo-electron microscopy (cryo-EM) in the 1980s. In cryo-EM, proteins are flash-frozen in their native states and imaged using an electron microscope with enough resolution to capture large protein complexes and membrane proteins. Both techniques fed their respective outputs into a growing open-science data repository called the Protein Data Bank (PDB). Launched with just seven structures in 1971, the PDB is a digital archive that stores the precise three-dimensional coordinates of every atom in a protein structure, along with experimental details about how those coordinates were determined.
Yet even with the steady advancement of laboratory tools like cryo-EM and data from the PDB, determining a single protein structure still took months or years of work. By 2020, after more than a half-century of effort, scientists had solved only about 170,000 protein structures — a tiny fraction of the millions known.
This all changed in 2021 when John Jumper and Demis Hassabis, a team at Google DeepMind, debuted AlphaFold2. Trained on data from the PDB, this system could predict highly accurate protein structures in a matter of minutes. In the Critical Assessment of Structure Prediction (CASP) competition, where methods are evaluated on their ability to predict the atomic coordinates of recently solved structures, AlphaFold2 achieved a level of precision comparable to experimental techniques for the majority of the proteins it saw. This was an unprecedented leap, leading the competition's founder John Moult to declare that the protein structure prediction problem was “in some sense solved.”
Indeed, within two years of AlphaFold’s release, it had been used to predict structures for the human proteome as well as many other biologically relevant proteins — over 200 million structures in total. Computational biochemist and 2024 Nobel Laureate David Baker and his team at the University of Washington followed shortly after with RoseTTAFold, a similarly-trained structure prediction model. Structural biology quickly moved from a bottlenecked experimental science to one where any researcher could easily access the likely shape of almost any protein.
The states are considered “likely” because these AI tools have significant blind spots. While AlphaFold and similar tools excel at predicting a protein's most stable form, they fail to predict how proteins “wiggle” and “shake” between multiple structures. Inside living cells, proteins behave more akin to liquids than solids, twisting and reshaping themselves as they carry out functions. Enzymes, for example, dramatically change shape to grab onto their target molecules. Molecular machines like kinesin walk along cellular tracks by cycling through different conformations. Signaling proteins switch between active and inactive states. These dynamic processes — the very essence of how proteins actually work — remain largely invisible to current prediction tools.
The problem lies in how these AI systems were trained. For each protein structure in the PDB, AlphaFold2 is shown the corresponding protein sequence (as well as evolutionarily related protein sequences) and tasked with predicting the 3D coordinates of each atom. Through this process, the model learns to predict the most likely structure for a given protein, typically representing a single thermodynamically favorable conformation. While this training paradigm has produced incredibly powerful models, its inability to predict anything but these most likely structures can flatten the functional diversity of the protein world under study.

The limitations of this training paradigm are especially apparent when trying to predict the structures of proteins that change shape as part of their function — a property that appears to be significantly more common than the single states generated from AlphaFold or cryo-EM alone suggest.
Take ATP synthase, the molecular motor that creates fuel molecules for the cell. We know from direct observation that it functions like a nanoscale rotary engine, with its central shaft spinning as it converts energy from proton flow into ATP production. While AI models can predict the shape of individual ATP synthase components with impressive accuracy, they can't capture the dynamic rotary motion because the data fed into these models only show the most easily crystalized protein conformations. The same shortcoming applies to membrane proteins, which must flex and bend to transport molecules across cell membranes.
While efforts to shift towards developing models that can predict protein flexibility and conformational changes are underway, progress has been slow. Attempts to adapt AlphaFold2 to predict multiple state conformers, for example, have only achieved limited success.
This suggests that researchers stand at a crucial crossroads in structural biology, in need of not just better computational tools but entirely new model systems for studying protein dynamics.
Prions for Protein Dynamics
Prions offer a way forward. Their ability to switch between distinct stable states — from soluble monomers to aggregated polymers — represents one example of dramatic protein conformational change that can be extrapolated to understand how proteins fold and interact more broadly.
The prion transition provides generalizable insights; it demonstrates how local interactions between protein domains can trigger global conformational changes, and it showcases how relatively small changes in amino acid contacts can lead to dramatically different stable states with altered biochemical properties. These act as observable readouts in model organisms like yeast.
Beyond protein dynamics, prions also reveal mechanisms by which conformational changes in properly coded proteins can lead to dysfunction without genetic mutation. This, in turn, helps expose the relationship between a gene’s code and the protein that it ultimately blueprints for.
Recent advances in cryo-EM have made it possible to capture high-resolution structures of prion proteins in both their normal and misfolded states, as well as intermediate forms during the conversion process. By flash-freezing prions at different stages of their transformation, scientists can create a molecular timeline that shows how these proteins morph from one state to the next.
Despite these promising avenues, prions remain underutilized in the broader study of protein dynamics, partly due to safety concerns. They are deadly, after all. Pathogenic prions cause fatal neurodegenerative diseases with 100 percent mortality rates, and unlike bacteria or viruses, they can't be neutralized with standard sterilization techniques.
Fortunately, the discovery of beneficial prions in yeast offers a safer path. These proteins exhibit similar conformational switching and self-propagation as their dangerous human counterparts but in a benign, easily manipulated organism. Beyond benign yeast models, modern biosafety protocols and specialized Level 3 containment facilities allow for the safe study of pathogenic prions, while computational modeling and in vitro systems offer additional routes to understanding prion behavior without exposure risks.
If AI models can learn to predict how and when prions switch shapes and influence other proteins to do the same, the lofty goal of solving the protein folding problem will be that much closer. Given recent evidence that many proteins in the PDB actually exist in multiple stable forms rather than just one fixed shape, this seems especially relevant. According to this view, prions aren’t outliers but rather dramatic examples of what many proteins do more subtly.
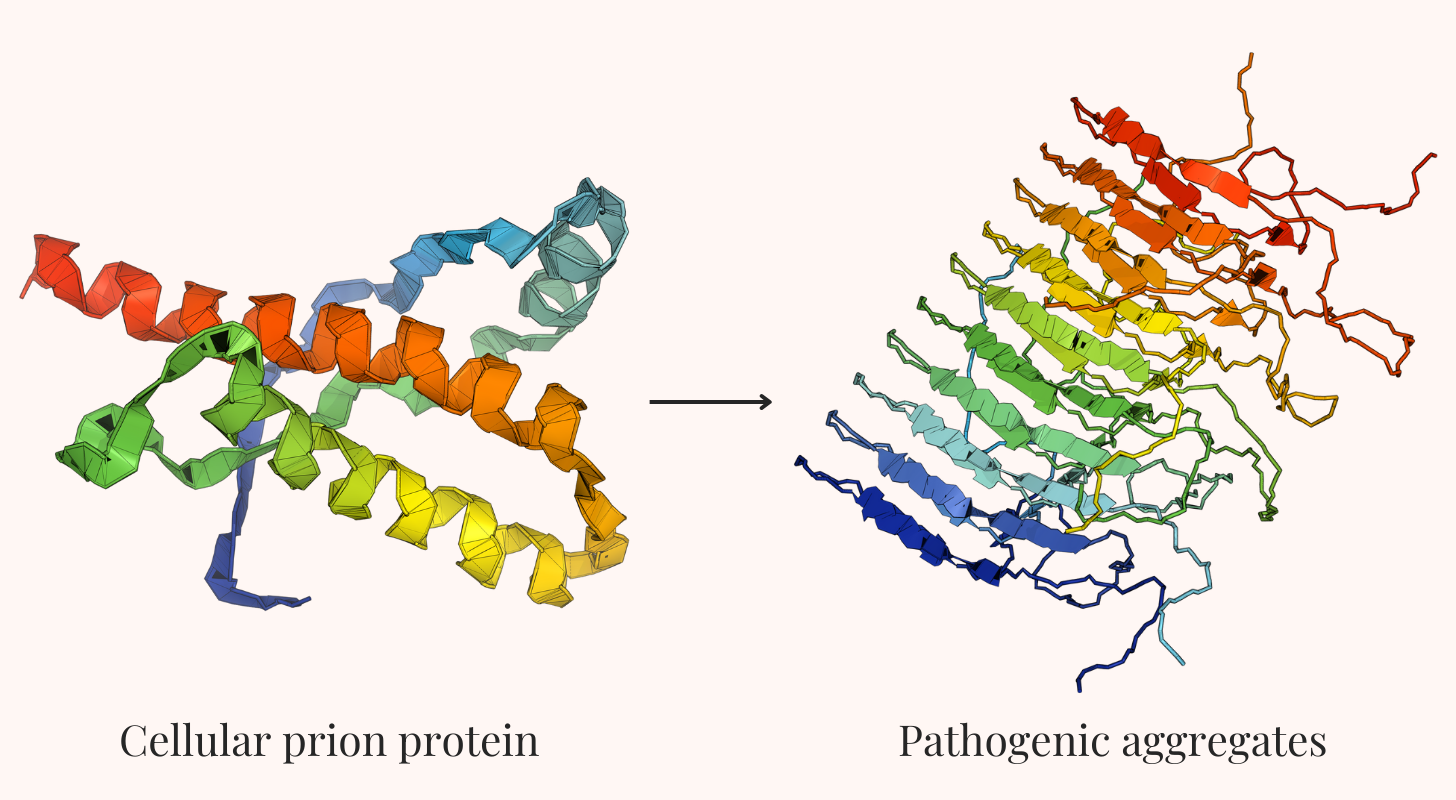
Studying prion shape-shifting could also unveil a more fundamental feature of natural, enzymatic machinery. Enzymes are, by definition, functional proteins that impose their will through changes in structure. It follows, then, that studying prions might point towards principles applicable to the design of other functional and helpful proteins custom-built for any task.
Just like E. coli’s use case extends beyond learning about the lac operon and into a useful tool for biological applications through transformation, prions have the potential to serve as more than a model for protein dynamics. Studies on functionalized prion-inspired amyloids, for example, have shown promise in biosensor applications, where the controlled aggregation properties of these proteins enable novel detection mechanisms. Similarly, research into prion protein-ligand interactions has revealed how small molecules can stabilize particular conformational states, potentially informing broader strategies for controlling protein shape. And, again, prions might be able to reveal the mechanisms of neurological decline.
Prion research even has the potential to reveal some greater unknown. Studying bacteria revealed transformation (a method to push DNA into living cells) and DNA polymerase. Studying viruses illuminated reverse transcriptase, obtained incidentally while in pursuit of other discoveries. Studying fungi led to antibiotics — probably the single greatest life-saver in the 20th century. History suggests that the investigation of distinct biological phenomena often leads to the inadvertent discovery of tools that transform our ability to manipulate living systems. Prion study could enable synthetic biology’s dream of making a protein for every purpose.
The implications of such an achievement would extend far beyond academic interest: The challenge of designing stable protein therapeutics for neurological conditions like Alzheimer’s, where current antibody treatments struggle to cross the blood-brain barrier or maintain structure long enough to be effective, could be overcome by discovering principles of protein design drawn directly from prions. Even industrial challenges, like making enzymes that could break down plastic waste or capture carbon dioxide more efficiently than natural proteins, will rely on our ability to understand, predict, and ultimately dictate protein dynamics.
Back in 1999, Susan Lindquist stated: “[protein] studies in yeast provide an opportunity to greatly accelerate the rate at which we can probe the fundamental nature of human disease processes and design and test new therapeutic strategies. Fundamental principles of biochemistry and cell biology unite the diverse forms of life on Earth.” The twenty-six intervening years, in which we have gained a deeper understanding of the workings of prions, have brought us ever closer to such a future.
{{divider}}
Eryney Marrogi is a medical student at the University of Vermont, with experience in biological engineering from working on mosquitoes at Harvard, AAV at Dyno Therapeutics, and novel biosensors at Caltech. His work in synthetic biology and medicine extends to writing about biological innovations on his Substack.
Theo Sternlieb is a research scientist at Dyno Therapeutics where he works on generative modeling for AAV engineering. His interests include structural biology, deep learning and music.
Cite: Marrogi, E. & Sternlieb T. “How Prions Can Unlock Protein Design.” Asimov Press. DOI: 10.62211/54tr-21po
Video: 263K prions were isolated from an infected hamster brain and then imaged using cryo-EM. Credit: Kraus A. et al. Molecular Cell (2021).
Lead image by Ella Watkins-Dulaney.
Thank you to Stephen Malina, Max Berry, Neal Nolan, Shelby Newsad, Effie Klimi and Andrew Warfield for their comments on this piece.
Always free. No ads. Richly storied.
Always free. No ads. Richly storied.
Always free. No ads. Richly storied.