Defense-Forward Biosecurity
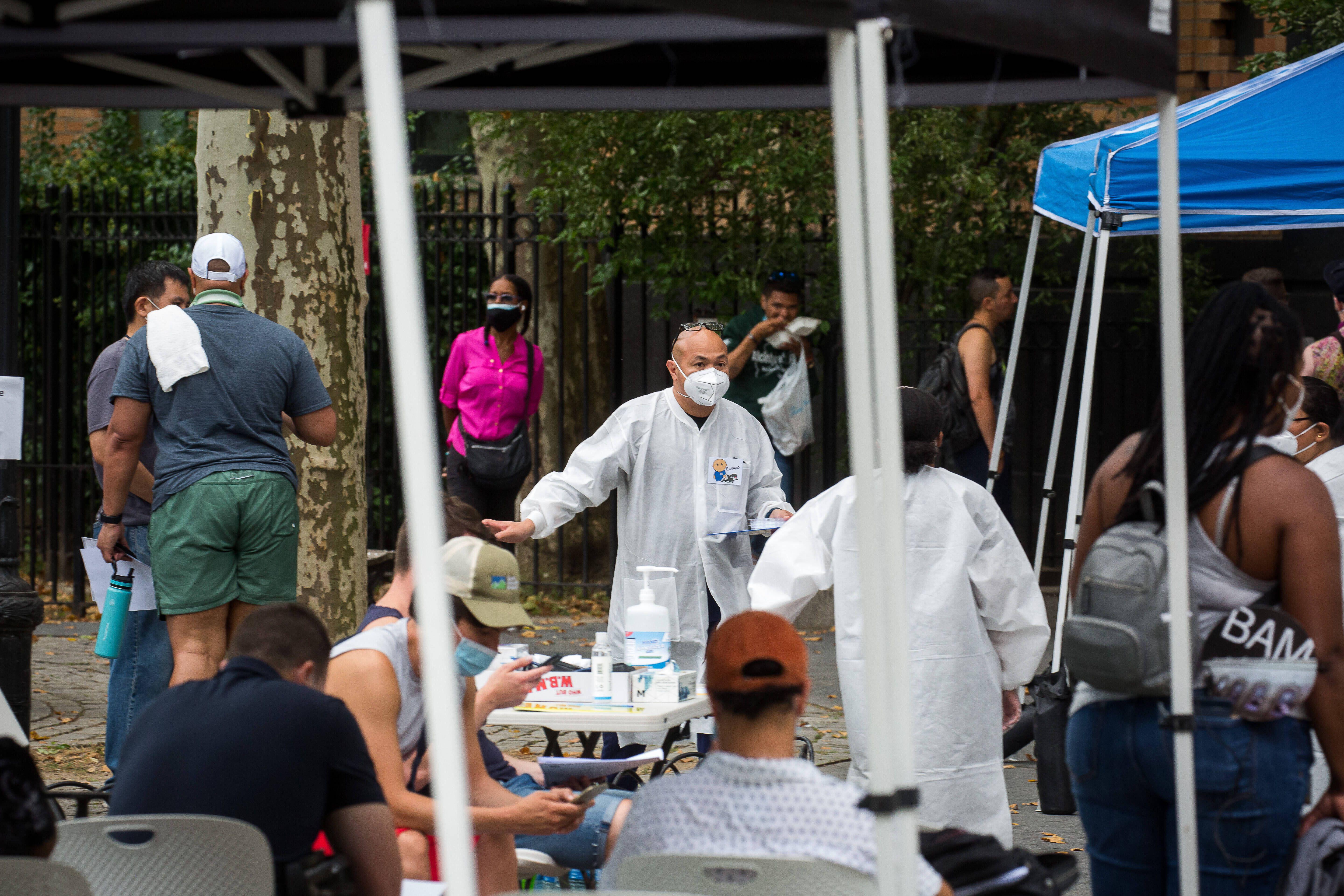
Doctors in training are told that when they hear hoofbeats, they should think horses, not zebras; rare diseases are the exception, not the rule. Sometimes, though, novel diseases do emerge, and as COVID-19 demonstrated, they can surprise us. Identifying a new or unexpected pathogen quickly, particularly when it occurs in conjunction with other, more common illnesses, is a key challenge for doctors, researchers, and public health professionals concerned with disease preparedness and biosecurity.
Consider Alice, a 49-year-old woman (and hypothetical patient) who walked into a hospital in Puerto Rico with joint pain, a headache, nausea, and a low fever. She had recently traveled to Brazil to visit relatives and had felt achy for the past two months, beginning a few days after she returned. Painkillers and anti-inflammatories did not alleviate her symptoms. Her blood cell counts were low, but her hemoglobin was normal and a urine culture found no signs of a bacterial infection.
When doctors sequenced the DNA and RNA found in Alice’s blood and synovial fluid—the liquid that surrounds and lubricates joints—they found abnormally low levels of genes encoding iron-storing proteins and high levels of epidermal growth factor receptor RNA. The results suggested a dual infection; Chikungunya and Zika, both of which circulate in Brazil.
While Alice was lucky that her infections were identified at the hospital, it would have been even better had they been identified before she arrived.
A “holy grail” of biosecurity has long been to build a continuous, real-time biosurveillance system that can detect pathogens circulating in airports, train stations, or other public places before they cause a public health crisis. Illustrating this, the Apollo Program for Biodefense, part of a bipartisan commission co-chaired by former Senator Joe Lieberman and former Secretary of Homeland Security Tom Ridge, recently called for “Ubiquitous Sequencing” as a key part of its strategy to detect future outbreaks.
Unfortunately, sequencing technologies have historically been difficult to implement at scale. It is expensive and time-consuming to swab, sequence, and interpret biological data. But as sequencing technologies become smaller and cheaper, and AI tools are trained to process data with lower error rates, we are hurtling toward an inflection point. In the next five years, these technologies can enable us to build a global network of biosurveillance stations—but only if we choose to make it happen.
{{signup}}
Reading DNA
The first step in detecting a novel pathogen is recognizing it as an anomaly amidst a noisy background of other material. Filtering out just the DNA from a messy sample and sequencing it isn’t a new technique; but doing so quickly and in large quantities are, in essence, the elements that make sequencing usable at scale.
Massively parallel sequencing, or “next-generation” sequencing, involves making many copies of the DNA strands found in a sample by using “universal primers.” These are short sequences of DNA that are highly conserved, meaning they are likely to be present in a wide variety of sequences. These primers bind wherever they can, and then polymerase chain reaction, or PCR, is used to amplify every sequence attached to the primers. After copying the DNA to form a big pool, each piece is sequenced.
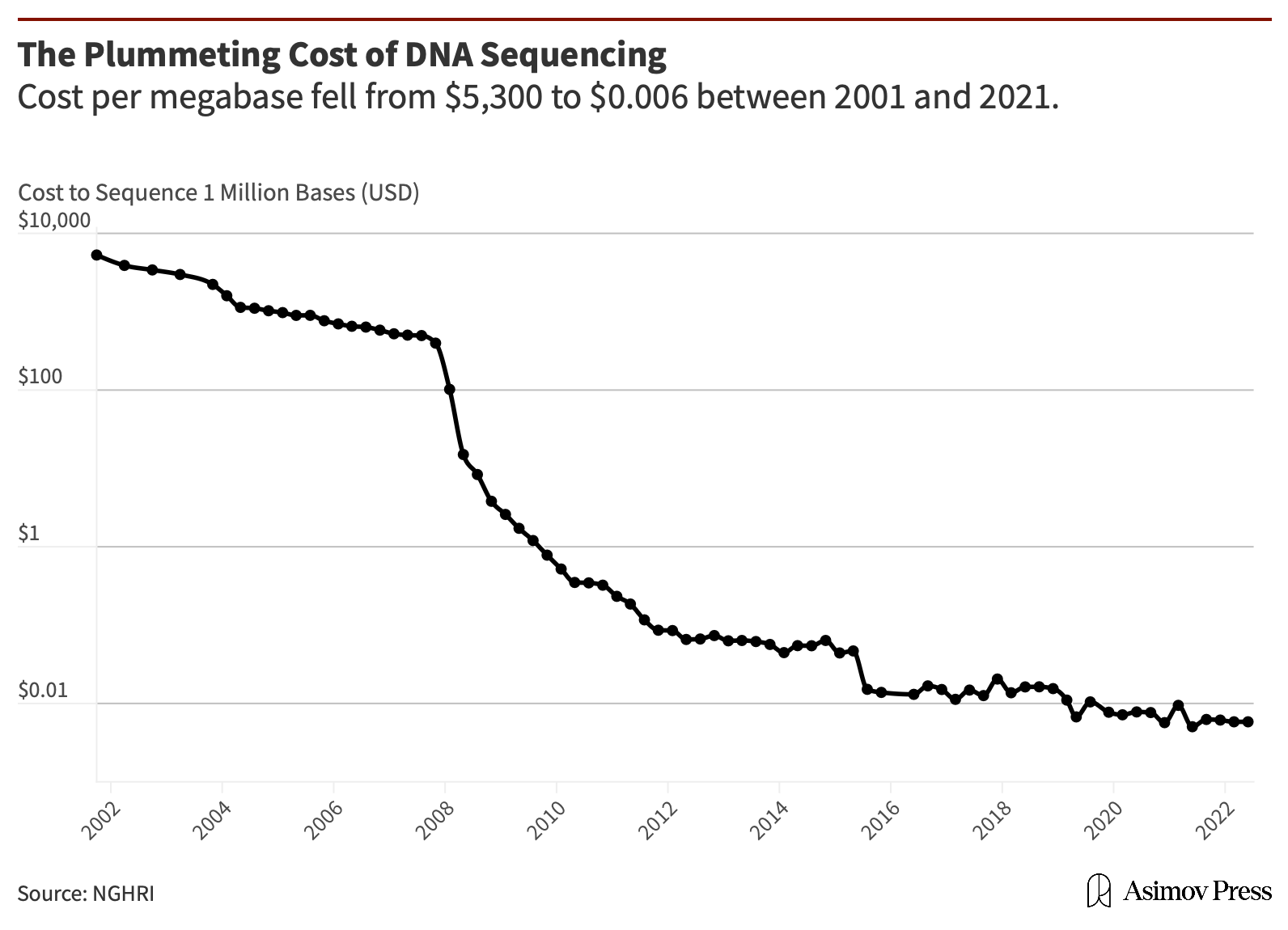
This process of sequencing everything in a sample and sorting the results later is called metagenomic sequencing because it focuses on making one large library of genomic data—a “meta” genome—before assembling each gene fragment into the genomes belonging to individual organisms.
While reading all the sequences present in a sample, researchers want to find any that are out of place, such as those that belong to a never-before-seen virus. Irrelevant sequences are removed, and everything else is compared to a library of common viruses and bacteria or the Virulence Factor Database to find sequences that might indicate the presence of a pathogen. This cleaning, annotation, and characterization work is all performed computationally.
But DNA alone is not always enough to identify a pathogen. Extra pieces of data, including proteins, lipids, or small molecules, are helpful when a particular DNA sequence is difficult to amplify or isolate from a sample, or when sequencing results don’t point squarely at a single disease-causing organism. For example, many different filoviruses attack white blood cells and cause similar innate immune responses, which makes it difficult to identify a particular species from only their DNA.
In order for “multi-omics” analyses to work, though, we first need to know both the way an infection progresses and the effects it has on a patient. Initially, patient samples must be studied to build out a history of “training data” against which to compare data from new samples. The greater the variety of infections that have been previously sampled—encompassing many families of viruses, bacteria, fungi, and parasites—the greater the likelihood that a new infection will be detected.
Real-Time Detection
Biosurveillance networks, built upon metagenomics and other tools, are becoming increasingly feasible. The U.S. government already deploys biosurveillance technologies and funds the development of new sequencing platforms. The Department of Homeland Security (DHS) has rolled out surveillance tools to look for target pathogens on a priority list (like the Select Agent list, which outlines microbes, viruses, and toxins with “the potential to pose a severe threat to both human and animal health.”) But a more agnostic approach will be useful to detect emerging pathogens, as was the case during the initial spread of SARS-CoV-2.
In 2003, the DHS launched the BioWatch program after five people died and twenty fell ill from touching envelopes laced with anthrax spores. The BioWatch program deployed air sampling devices at airports, stadiums, and other highly trafficked areas in 30 cities. The initial BioWatch machines required off-site laboratory analysis, which means a trained technician had to go to each machine, collect samples, and then study those samples in a laboratory. Newer BioWatch machines, though, collect pathogens from the air and analyze them autonomously, thus decreasing the time gap between exposure and detection. The machines use an anomaly-detection algorithm that picks up signals during environmental sampling that may indicate a hazard—a higher-than-normal number of particles, or particles that are a certain size, charge, or molecular weight. When an anomaly is detected, a technician comes to remove the filter and analyze the data.
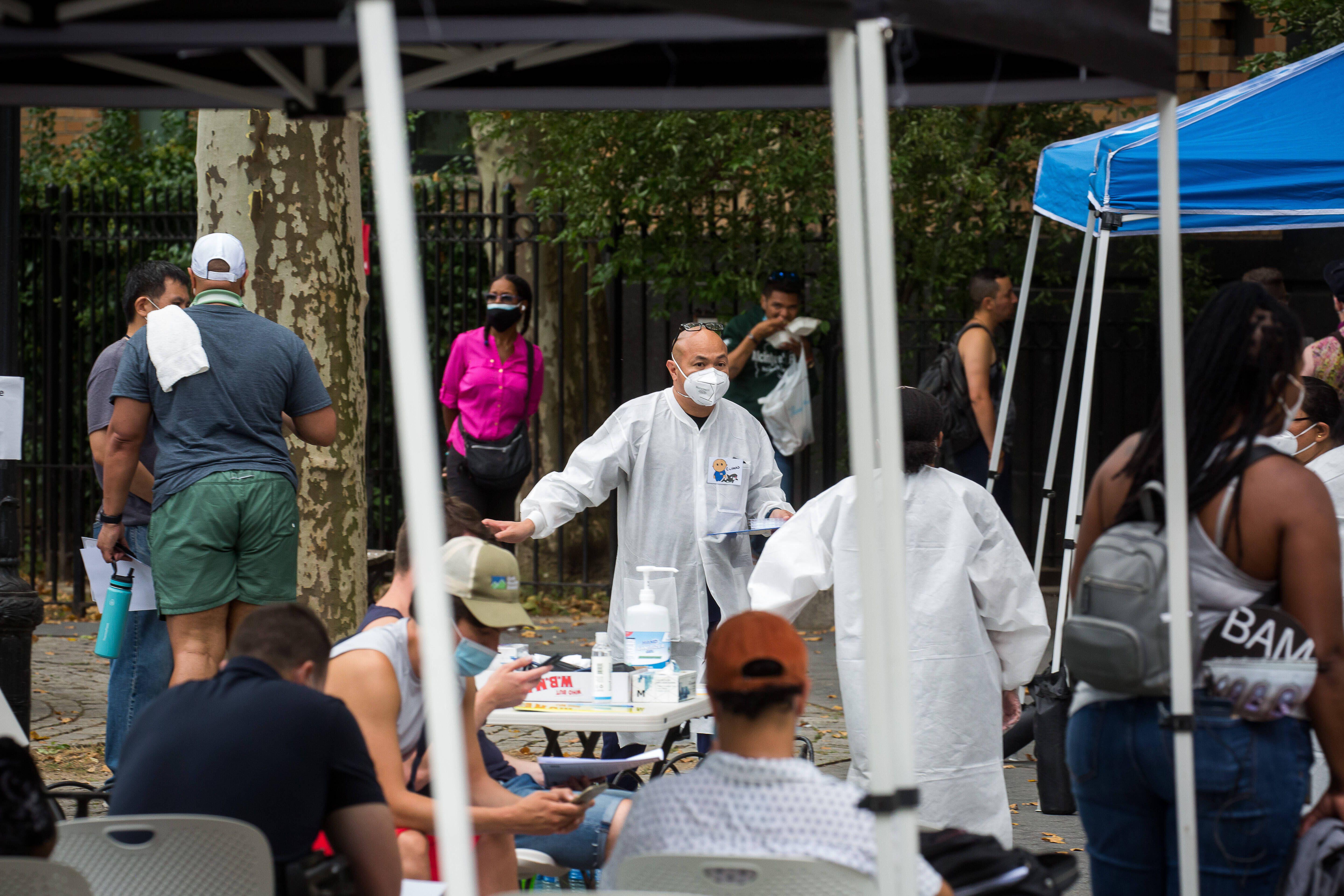
A new pathogen can emerge anywhere in the world, though, so disease surveillance and monitoring should ideally happen in cooperation with other countries. Since 1997, the Department of Defense has operated the Global Emerging Infections Surveillance Program. At least nine laboratories outside the U.S. are part of this program, and use next-generation sequencing to analyze samples collected from hospitals, clinics, veterinary facilities, or specific environmental locations. These laboratories share data and bioinformatics tools that enabled the early detection of SARS-CoV-2 and monkeypox even before their reference genomes were available. Federally funded laboratories must also deposit genetic sequences into public databases, such as GISAID and GenBank.
Despite a historical interest in detecting emerging pathogens, available technologies were simply too slow or expensive to roll out more broadly. But these technologies continue to improve along both axes.
Whereas early next-generation DNA sequencers took several days to sequence 50-100 gigabases (1x109 bases), newer machines can process the same amount of data in 1-2 hours and can sequence 16 terabases (1x1012 bases) in 48 hours. The accumulation of more data from more types of infections, and better computational tools to process and analyze that data, have increased the speed and accuracy of identifying pathogens.
AI tools are also a major catalyst for DNA sequencing, thanks to their uncanny ability to recognize and match patterns. An example of the application of AI to metagenomics is a tool that Lawrence Livermore National Laboratory recently released, an open-source Metagenomics Analysis Toolkit that can analyze any sequence against a library of every available sequenced genome from “viruses, bacteria, archaea, protozoa, fungi, and several variants of the human genome.” The toolkit uses clever computational techniques to improve the speed of sequence comparisons, comparing each one to a library of 25 billion sequences. Karius, a startup in Redwood City, California, also sells a test that detects and sequences cell-free microbial DNA in a blood sample and then uses AI tools to quickly match sequencing results to more than 1,000 different pathogens.
As promising as expanding metagenomic sequencing is though, it will still require reduced costs, faster sample processing, and a tighter integration of AI analysis tools. Metagenomic sequencing is still too expensive because of the large number of reads needed—related to the size of the sample—and because using sequencing effectively as a surveillance technique requires sampling frequently and from a variety of locations, which means more devices, more samples, and more technicians.
Putting a sequencer in every port, hospital, water treatment plant, or subway station isn’t feasible just yet due to the expense. But just as the costs of computing power decrease regularly with technological improvement, the costs of sequencing a given quantity of DNA continue to fall. Although reductions in the cost of DNA sequencing have slowed in the last few years (when evaluated against earlier drops in cost, such as after the introduction of parallel sequencing, in 2007, and simultaneous double-stranded nanopore sequencing, in 2015). Currently, reductions in cost are due to improvements in the size and configuration of chips, which, similar to how Moore’s law predicts reductions in the cost of computing power, means we have cause to be hopeful. The next 5-10 years could see sequencing costs cut in half thanks to more robust reagents, better chips, and smaller channels to fit more sequencing reactions into the same amount of space on those chips.
Today, individual machines cost between $350,000 and $985,000. The MinION nanopore sequencer is much more cost-effective, at $1,999 for the device and reagents, with a cost per run of about $150-$400 depending on the sample size. Sample preparation kits and reagents are also prohibitively expensive for labs in under-resourced areas, where there is often a dearth of trained technicians who can service machines or provide repairs.
Nevertheless, the costs of DNA sequencing have dropped since 2001, and since 2008 have decreased at a pace more rapid than that which Moore’s Law predicts for computing power. The cost of sequencing a human genome is now less than $1,000, down from $3 billion to sequence the first one, and the cost of a megabase of DNA (one million bases) has fallen to below a penny. These favorable cost trends have made thinkable, and increasingly available, the solution of sequencing the trillions of bases present in a sample of drinking water, say, to find the few Cholera genomes that would indicate contamination.

Large-scale metagenomics, in public places, is not yet cost-effective. But the economics already make sense at a smaller scale, such as in hospitals. Sequencing a single patient sample, including all the collection, preparation, and data processing, costs about $350-$700. That price tag seems worthwhile if the sequencing data leads to earlier treatment, faster recovery to productivity, and ultimately lives saved. The current cost and turnaround time, though, means that physicians typically start their diagnoses by running tests for particular pathogens first (at a cost of around $50 per sample) and only turn to metagenomic sequencing when, for example, a patient doesn’t respond to broad-spectrum antibiotics.
There is hope in further reducing sequencing costs, though, one of which is to minimize the amount of DNA that must be sequenced to begin with. A 2021 meta-analysis of thirteen studies found that, on average, about 90 percent of the sequencing reads produced from a sample are just human DNA, even if the sample has been purified to remove human DNA. If we get better at purifying samples, then, and use positive and negative controls to remove irrelevant or contaminated sequences, metagenomic sequencing could (hypothetically) fall ten times in price.
Sequence Everything
The outlook for pathogen-agnostic sequencing is buoyed by three trends: the combination of falling costs of metagenomic and multi-omic tools, a “defense forward” approach to public health and increased interest in biosurveillance, and the development of AI tools to sort through the massive amounts of data that these techniques generate. If these trends continue, the next decade should see an increase in disease surveillance that is faster, cheaper, and more prevalent.
We are likely to see this in the form of metagenomic sampling systems that rely on portable, automated devices for DNA extraction and sequencing. These future systems could take many forms. For example, they could look something like BioFire’s closed detection system, coupled with either on-board computing power to analyze those results, or the system developed by Spanish researchers to conduct on-site sequencing in the Canary Islands, or a link to a cloud laboratory analysis provider like CZ ID, which has a fully automated pipeline to analyze raw sequencer data and return results in hours, for free or at very low cost. On the whole, the costs of these systems are still too high to be widely deployed (the BioFire system costs about $36,000), but price trends are encouraging, especially as pilot programs prove their efficacy and value.

Pipelines like these, which couple portable sequencers with computational tools to rapidly identify rare or emerging pathogens, are already being used in low-resource or high-disease-burden settings, like Bangladesh and Cambodia.
For example, Cambodia has had 11 human cases of H5N1 avian influenza in the past year, a rate of cases that the director of the Cambodian Center for Disease Control attributes to an increase in sample testing driven by sequencing. This speedy and well-connected surveillance network grew out of changes made between 2002 and 2006 when USAID and the U.S. CDC helped Cambodia respond to a previous avian influenza outbreak. Lacking national public health infrastructure at the time, Cambodia had been sending its samples to France, which delayed results by a month. Thanks to the aid, the Cambodian CDC improved its testing and reporting capabilities, and now tests samples for free, reports out to other labs and hospitals in Cambodia within hours of a confirmed case, and receives samples from hospitals and agricultural inspectors who are required to send samples from patients with severe respiratory illness. While there is room to automate this system even further, there have been huge strides.
The benefits of increased sequencing and testing are faster response to cases, a better picture of how cases are spreading across the country, and strong partnerships with both local hospitals and international public health organizations that allow responses to be scaled up or targeted as needed. Globally, clinicians and public health workers are using sequencing-based surveillance systems to identify novel pathogens and local or differentiated strains of viruses that are causing difficult-to-treat illnesses. Even analyzing samples taken from patients without symptoms provides useful information about regional pathogen landscapes, the circulating prevalence of viruses, and rates of asymptomatic infection.
Should the deployment of these localized biosurveillance systems continue and expand, then the coordination of a worldwide pathogen-agnostic biosurveillance system becomes increasingly likely. With the help of a coordinating federal agency, such as the CDC, to combine and interpret the data from environmental surveillance and metagenomic sequencing as well as from hospitals using multi-omic and metagenomic approaches to identifying disease, responses to novel threats can be quickly initiated and directed.
If a monitoring device in a Cambodian jungle detects a newly emerging strain of typhoid, the right antibiotics can be identified and shipped to local health providers. If hospitals in Colorado report patients with asymptomatic infections from a new tick-borne illness, public health departments can warn local residents while scientists get to work developing treatments. If a new mutation in seasonal flu is detected in the wastewater of flights from Heathrow, a vaccine effective against that mutant strain can be prioritized for Londoners, and travelers can be screened for flu symptoms before boarding.
The first cases of COVID emerged, undetected or under-addressed, several months before pandemic response measures were enacted in March 2020. We lost valuable time. But with the kind of early warning that a defense-forward biosecurity network would enable, we can respond more effectively and urgently to future threats.
{{divider}}
Dr. Allison Berke is the Chemical and Biological Weapons Non-Proliferation Program Director at the Middlebury Institute of International Studies in Monterey, California. Previously, she was the Director of Advanced Technology at Stanford’s Institute for Economic Policy Research.
Cite: Allison Berke. “Defense-Forward Biosecurity.” Asimov Press (2024). DOI: https://doi.org/10.62211/25tk-28dj
This article was published on August 27, 2024.
Always free. No ads. Richly storied.
Always free. No ads. Richly storied.
Always free. No ads. Richly storied.