AI-Designed Enzymes
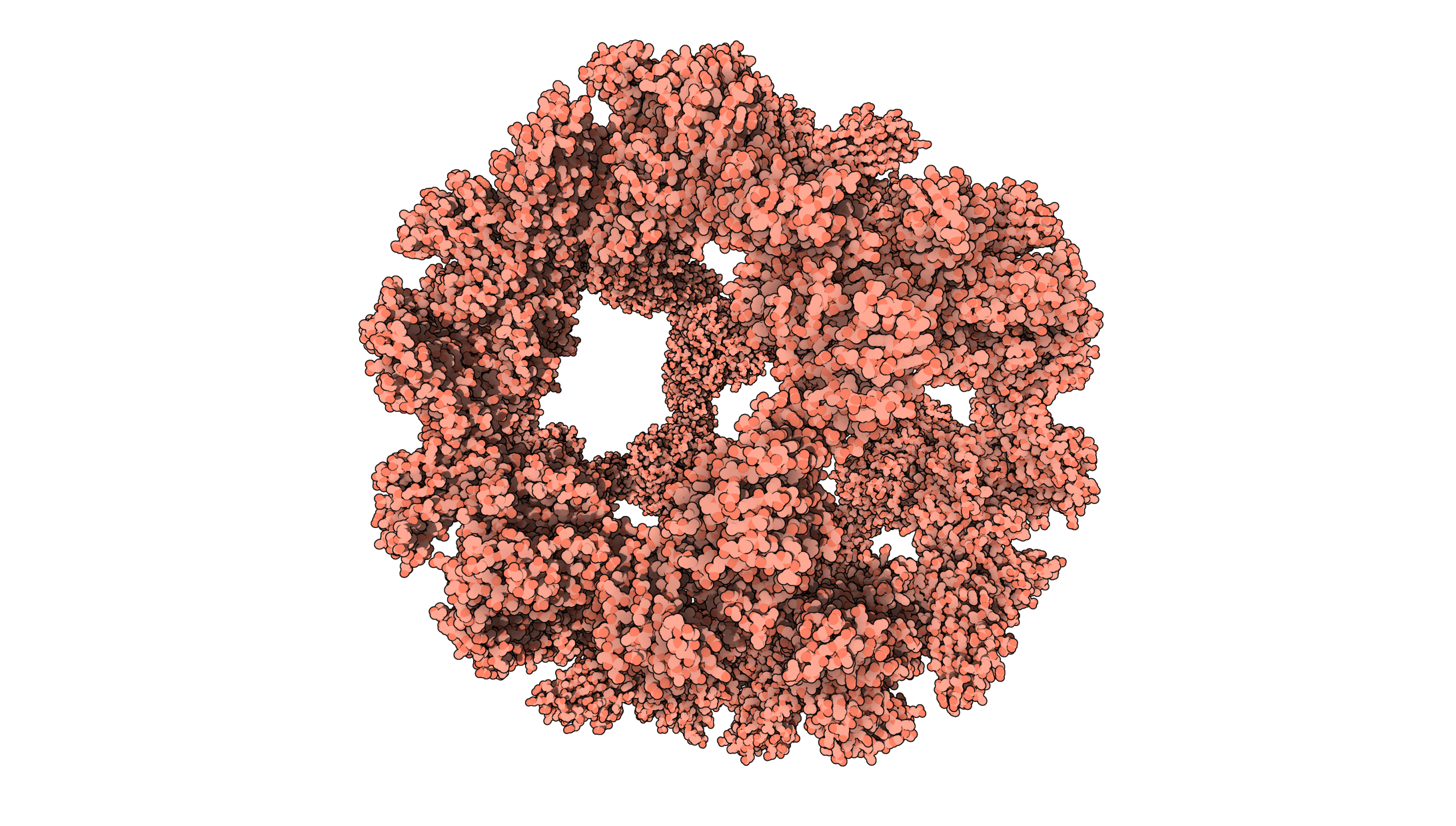
One of the ultimate aims of protein design has long been to build entirely new enzymes — proteins that perform specific chemical reactions — from scratch. These molecular machines power various cellular processes, from converting sugar into energy to switching genes on and off. Both Cas9 and hydrolases (widely used in the dairy and laundry industries) are also enzymes.
Traditionally, scientists discover enzymes in nature and adapt them through trial and error. But what if, instead, researchers could create bespoke enzymes from the ground up?
Despite recent advances in AI, doing so remains a formidable challenge. Enzymes often change shape dramatically while catalyzing reactions. However, most protein design methods focus on static structures, treating proteins as fixed rather than dynamic, fluid entities. This is because the protein structural databases used to train these models consist overwhelmingly of structures gleaned from protein crystals or other “frozen” images. The resultant distortion has made computational enzyme design exceptionally difficult.
A new paper, published today in Science, suggests a way forward.
David Baker’s group at the Institute for Protein Design has developed a method to computationally design serine hydrolases, a class of enzymes involved in everything from blood clotting, digestion, and nerve signaling. Their study not only demonstrates the feasibility of designing this class of enzymes, but also provides a roadmap for creating other types of dynamic, moving proteins.
{{signup}}
The Challenge
The principles of modern enzyme design trace back to a fundamental insight gleaned by Bill DeGrado and others in the 1980s: simply positioning amino acids to mimic natural enzyme active sites produces catalysts that are thousands to millions of times slower than their natural counterparts. The issue in enzyme design, then, isn’t so much about getting initial positioning of the reactive groups, but orchestrating a sequence of structural shifts needed for catalysis: guiding substrates into place, stabilizing intermediate states, and releasing products efficiently.
Despite the enormity of the problem, there have been plenty of prior attempts to design dynamic enzymes. One study from 2008 (also from the Baker lab) reported de novo enzyme catalysts for Kemp elimination — a reaction that opens up a benzisoxazole ring — by running molecular dynamics simulations to sieve through possible designs. For a more recent paper, scientists used a language model called ZymCTRL to create carbonic anhydrase and lactate dehydrogenase enzymes.
So what makes this new paper important?
Well, the enzymes designed in earlier studies were relatively simple; they catalyzed reactions with only one or two chemical steps, remained largely rigid during the reaction, and produced no intermediate byproducts. While many useful enzymes are similarly simple, most others are far more complex and have, until now, resisted computational design.
Serine hydrolases belong to this latter category. Their active site has three core amino acids — a serine, histidine, and aspartate — that work together with water to break ester bonds in a six-step process:
- A substrate binds to the enzyme’s catalytic site;
- The enzyme is modified into an intermediate form;
- A product is released;
- A second substrate binds to the enzyme’s catalytic site;
- A second product is released;
- The enzyme is restored to its original form.
In steps 1 to 3, the serine amino acid attacks the substrate, forming a stable intermediate called an acyl-enzyme. This releases an alcohol but leaves the enzyme in a modified state. In steps 4 to 6, a water molecule activated by the histidine attacks the intermediate, releasing an acid and restoring the enzyme to its original form. This multi-step process, with its intermediate states and byproducts, makes serine hydrolases particularly challenging to design. The enzyme must not only bind substrates correctly but also maintain stability through each transformation while handling intermediate products without inhibition.
Computational Design
To design a new serine hydrolase enzyme, Baker’s team turned to RFDiffusion, a protein design tool developed in their lab in 2022. RFDiffusion is an AI model that generates new data by learning from existing patterns — it’s like a Swiss Army knife for protein design. The researchers generated 10,000 potential designs for serine hydrolases, each built around a fixed catalytic site composed of serine, histidine, and aspartate.
In order to identify which of these 10,000 designs might function as actual serine hydrolase enzymes, the team used AlphaFold2 to predict protein structures, checking whether the anticipated structure of each design matched the one generated by RFDiffusion. While this step didn’t address the full complexity of enzyme function, it provided a quick initial filter. Unfortunately, the results were disappointing: only 1.6 percent of the designs showed any catalytic activity upon testing in the lab (more on their methodology later).
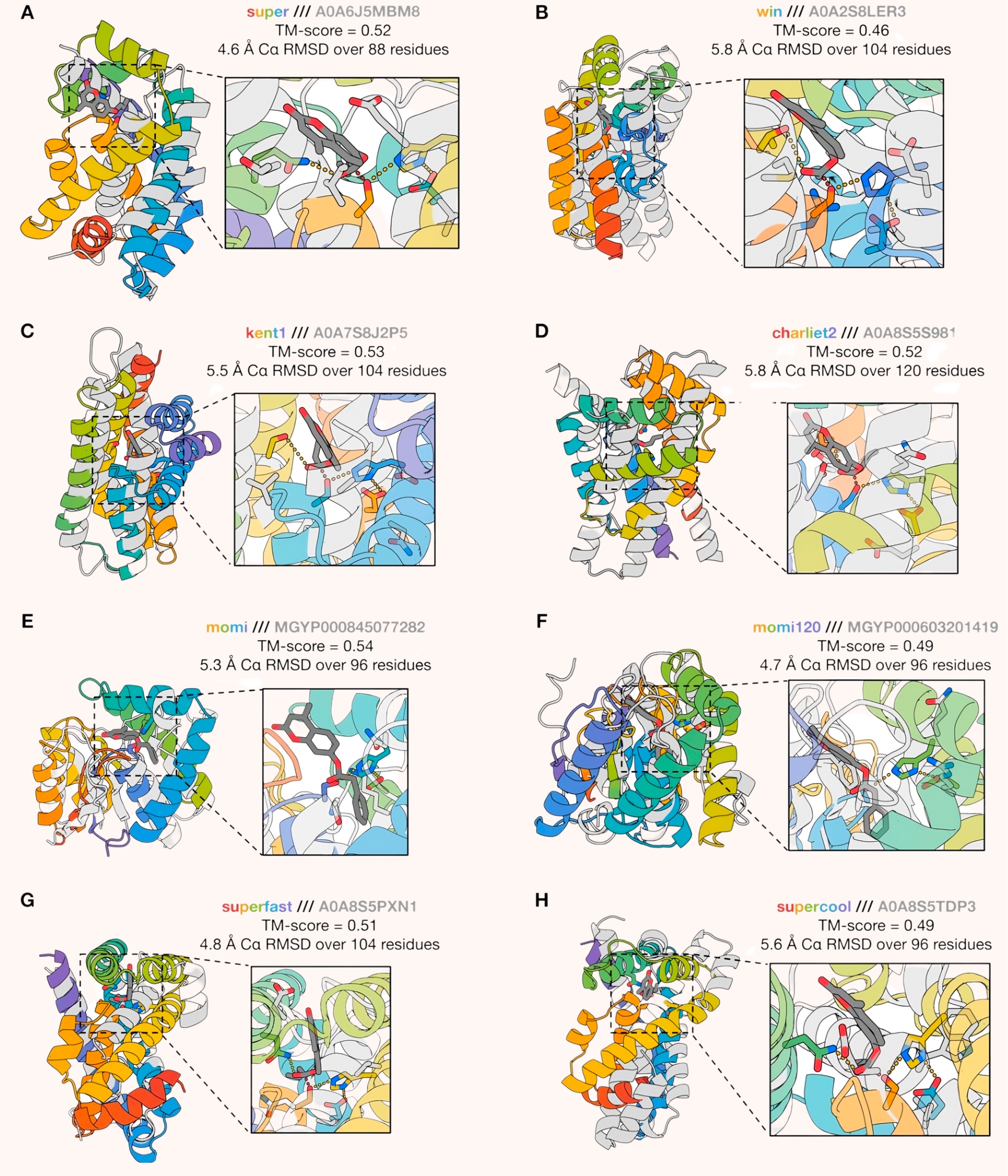
For a second round of filtering, the team introduced a new method called PLACER (Protein- Ligand Atomistic Conformational Ensemble Resolver), which predicts how atoms should arrange themselves based on physical and chemical principles.
By training PLACER on structures stored in the Protein Data Bank, the team taught the model to predict atom arrangements that follow the physical and chemical rules governing protein-small molecule interactions. For example, PLACER learned what hydrogen bond geometries are valid, how protein sidechains typically pack together, and which rotamer (or sidechain orientation) combinations are physically plausible. During testing, the team input a chemical structure into PLACER, which then generated about 50 possible outputs. The researchers evaluated these outputs to see if PLACER had added chemically reasonable features. If the additions looked correct, they "trusted the design.
During this second round, the team used PLACER to filter designs but only examined the apo state — the enzyme’s structure without any bound molecules. Specifically, they checked whether a hydrogen bond consistently formed between serine and histidine in PLACER’s predictions. If not, they discarded the design. This single change boosted performance four-fold: 5.2 percent of the enzymes now showed catalytic activity. However, these enzymes still got stuck after the first reaction cycle, forming an acyl-enzyme intermediate but failing to complete the full catalytic cycle.
For the third round, the team raised the filtering criteria further. They required designs to pass PLACER checks in both the apo state and the acyl-enzyme intermediate state, along with satisfying additional requirements for specific chemical features. This stricter filtering led to another leap in performance: 18 percent of the designs showed catalytic activity, and, crucially, two designs (1.6 percent) achieved multiple turnover catalysis, meaning they could complete the full catalytic cycle without getting stuck. This was a major milestone, as enzymes must typically catalyze reactions thousands to millions of times per second to be effective.
While natural serine hydrolases achieve turnover rates of 10²–10⁵ per second, the designed enzymes only managed rates of 10⁻³–10⁻¹ per second — quite slow, but still a significant step forward given the complexity of the task. Achieving a non-zero turnover rate at all is something that nobody has ever done before with enzymes as complex as these.
Lab Validation
After each computational design step, the researchers tested their designs in the lab. They synthesized DNA encoding each protein variant, expressed the proteins in E. coli, and used two fluorescence-based assays to evaluate function. One assay verified that the serine amino acid was properly activated using a fluorophosphonate (FP) probe, while the other measured catalytic activity. This two-step screening, optimized for high-throughput 96-well plates, allowed them to test hundreds of designs and distinguish between enzymes that could perform a single reaction step versus those capable of full catalytic cycles.
Introducing these lab validation methods boosted success rates dramatically, from 3 percent of designs showing serine activation in round one to 84 percent in round three, with catalytic activity increasing from 1.6 percent to 18 percent. Most importantly, out of more than 100 designs tested in round three, two enzymes achieved multiple turnover catalysis.
To prove that their best protein variants were indeed the products of their intensive ML-guided design rounds, the team validated their most successful enzymes with high-resolution crystallography — something that often takes months or years for a single protein. They determined the atomic structures of both successful catalysts from their initial campaign, as well as several other improved variants. These crystal structures revealed active site geometries that matched the computational designs with atomic precision. The team also put a huge amount of work into discovering what caused failure modes in each of the three rounds, what was unique about the designed serine hydrolases that managed to continue catalyzing, and a lot more. (They experimentally-characterized 812 protein designs in total.)
While this work represents a major advance, there’s still room for improvement. The designed enzymes’ turnover rates remain far below those of natural enzymes. Additionally, the Baker group’s laborious process raises the question: Shouldn’t we aim for a model that can intuitively design multi-step enzymes without requiring so many curated filtering steps?
In the long term, yes, one should expect the field to move towards all-in-one solutions. However, for now, we have a promising process for generating some of the most complex enzymes on Earth, which could plausibly extend to new classes of enzymes altogether.
{{divider}}
Abhishaike Mahajan is a senior ML engineer at Dyno Therapeutics, a biotech startup working to create better adeno-associated viral vectors using AI. He also writes on a blog focused on the intersection of biology and AI at owlposting.com.
Eryney Marrogi is a medical student at the University of Vermont, with experience in biological engineering from working on mosquitoes at Harvard, AAV at Dyno Therapeutics, and novel biosensors at Caltech. His work in synthetic biology and medicine extends to writing about biological innovations on his Substack.
Cite: Mahajan A. & Marrogi E. “AI-Designed Enzymes.” Asimov Press. DOI: 10.62211/97ry-32pk
Always free. No ads. Richly storied.
Always free. No ads. Richly storied.
Always free. No ads. Richly storied.